Sampling involves selecting a subset of a population and drawing conclusions from that subset. How you sample and who you sample shapes what conclusions you are able to draw. Ultimately, this chapter focuses on questions about the who or the what that you want to be able to make claims about in your research. In the following sections, we’ll define sampling, discuss different types of sampling strategies, and consider how to judge the quality of samples as consumers and creators of social scientific research.
Chapter Outline
- 6.1 Basic concepts of sampling
- 6.2 Nonprobability sampling
- 6.3 Probability sampling
- 6.4 Critical thinking about sampling
Content Advisory
This chapter discusses or mentions the following topics: cancer, substance abuse, homelessness, anti-LGBTQ discrimination, mental health, sexually transmitted infections, and intimate partner violence.
6.1 Basic concepts of sampling
Learning Objectives
- Differentiate between populations, sampling frames, and samples
- Describe inclusion and exclusion criteria
- Explain recruitment of participants in a research project
Population
In social scientific research, a population is the cluster of people you are most interested in; it is often the “who” that you want to be able to say something about at the end of your study. Populations in research may be rather large, such as “the American people,” but they are usually less vague than that. For example, a large study for which the population of interest is more generally “the American people” will likely specify which American people, such as adults over the age of 18 or citizens or legal permanent residents it is examining.
It is quite rare for researchers to gather data from their entire population of interest. This might sound surprising or disappointing until you think about the kinds of research questions that social workers typically ask. For example, let’s say we wish to answer the following research question: “How does gender impact success in a batterer intervention program?” Would you expect to be able to collect data from all people in batterer intervention programs across all nations from all historical time periods? Unless you plan to make answering this research question your entire life’s work (and then some), your answer is probably a resounding no. So, what to do? Do you have to give up your research interest because you don’t have the time or resources to gather data from every single person of interest?
Absolutely not. Instead, researchers use a smaller sample that is intended to represent the population in their studies.
Sampling frames
An intermediate point between the overall population and the sample that is drawn for the research is called a sampling frame. A sampling frame is a list of people from which researchers draw a sample. But where do you find a sampling frame? Answering this question is one of the first steps in conducting human subjects research. Social work researchers must think about locations or groups in which their target population gathers or interacts. For example, a study on quality of care in nursing homes may choose a local nursing home because it’s easy to access. The sampling frame could be all of the patients at the nursing home. You would select your participants for your study from the list of patients at the nursing home. An administrator at the nursing home would give you a list with every resident’s name on it from which you would select your participants. If you decided to include more nursing homes in your study, then your sampling frame could be all of the patients at all of the nursing homes you included.
The nursing home example is perhaps an easy one. Let’s consider some more examples. Unlike nursing home patients, cancer survivors do not live in an enclosed location and may no longer receive treatment at a hospital or clinic. For social work researchers to reach participants, they may consider partnering with a support group that serves this population. Perhaps there is a support group at a local church in which survivors may cycle in and out based on need. Without a set list of people, your sampling frame would simply be the people who showed up to the support group on the nights you were there. In this case, you don’t start with an actual list; you have a hypothetical one. The sampling frame only comes into existence after you go to the support group and collect names.
More challenging still is recruiting people who are homeless, those with very low income, or people who belong to stigmatized groups. For example, a research study by Johnson and Johnson (2014) attempted to learn usage patterns of “bath salts,” or synthetic stimulants that are marketed as “legal highs.” Users of “bath salts” don’t often gather for meetings, and reaching out to individual treatment centers is unlikely to produce enough participants for a study as use of bath salts is rare. To reach participants, these researchers ingeniously used online discussion boards in which users of these drugs share information. Their sampling frame included everyone who participated in the online discussion boards during the time they collected data. Regardless of whether a sampling frame is easy or challenging, the first rule of sampling is: go where your participants are.
selecting study participants
Once you have a sampling frame, you need to identify a strategy for sampling participants. You will learn more about sampling strategies later in this chapter. At this point, it is helpful to realize that there may be some people in your sampling frame that you do not ultimately to enroll in your study. You may have certain characteristics or attributes that individuals must have if they participate in your study. These are known as inclusion and exclusion criteria. Inclusion criteria are the characteristics a person must possess in order to be included in your sample. If you were conducting a survey on LGBTQ discrimination at your agency, you might want to sample only clients who identify as LGBTQ. In that case, your inclusion criteria for your sample would be that individuals have to identify as LGBTQ. Comparably, exclusion criteria are characteristics that disqualify a person from being included in your sample. In the previous example, perhaps you are mainly interested in discrimination in the workplace and don’t want to focus on bullying in schools. You might exclude individuals who have not worked, who are currently enrolled in school, or might even set an age limit to people who are legal adults and exclude people who are less than 18 years old. Many times, exclusion criteria are often the mirror image of inclusion criteria. This would be the case if the inclusion criteria included being age 18 or older and the exclusion criteria included being less than 18 years old.
At this stage, you are ready to recruit your participants into your study. Recruitment refers to the process by which the researcher informs potential participants about the study and attempts to get them to participate. Recruitment comes in many different forms. If you have ever received a phone call asking for you to participate in a survey, someone has attempted to recruit you for their study. Perhaps you’ve seen print advertisements on buses, in student centers, or in a periodical. As you learn more about specific types of sampling, you can make sure your recruitment strategy makes sense with your sampling approach.
Sample
Once you recruit and enroll participants, you end up with a sample. A sample is the group of people you successfully recruit from your sampling frame to participate in your study. If you are a participant in a research project—answering survey questions, participating in interviews, etc.—you are part of the sample of that research project. Some social work research doesn’t use people at all. Instead of people, the elements selected for inclusion into a sample are documents, including client records, blog entries, or television shows. A researcher conducting this kind of analysis, described in detail in Chapter 10, still goes through the stages of sampling—identifying a sampling frame, applying inclusion criteria, and gathering the sample.
Applying sampling terms
Sampling terms can be a bit daunting at first. However, with some practice, they will become second nature. The process flows sequentially from figuring out your target population to thinking about where to find people from your target population to finding a sampling frame of people in your population to recruiting people from that list to be a part of your sample. Through the sampling process, you must consider where people in your target population are likely to be and how best to get their attention for your study. Sampling can be an easy process, like calling every 100th name from the phone book one afternoon, or challenging, like standing every day for a few weeks in an area in which people who are homeless gather for shelter. In either case, your goal is to recruit enough people who will participate in your study so you can learn about your population.
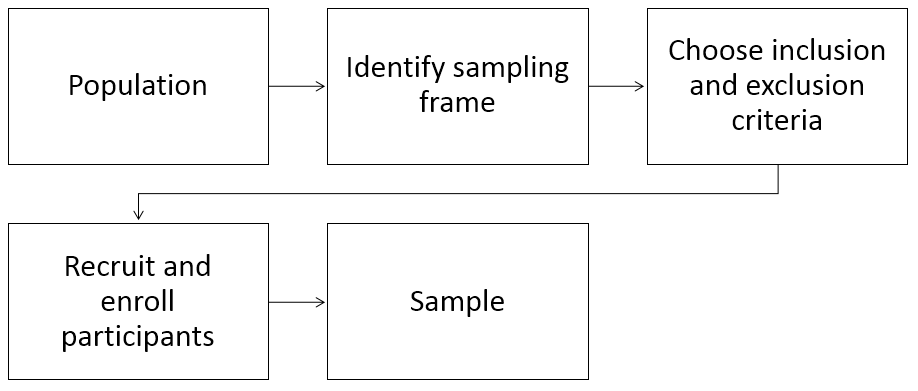
In the next two sections of this chapter, we will discuss sampling approaches, also known as sampling techniques or types of samples. Sampling approach determines how a researcher selects people from the sampling frame to recruit into her sample. Because the goals of qualitative and quantitative research differ, so too does the sampling approach. Quantitative approaches often allow researchers to make claims about populations that are much larger than their actual sample with a fair amount of confidence. Qualitative approaches are designed to allow researchers to make conclusions that are specific to one time, place, context, and group of people. We will review both of these approaches to sampling in the coming sections of this chapter. First, we examine sampling types and techniques used in qualitative research. After that, we’ll look at how sampling typically works in quantitative research.
Key Takeaways
- A population is the group who is the main focus of a researcher’s interest; a sample is the group from whom the researcher actually collects data.
- Sampling involves selecting the observations that you will analyze.
- To conduct sampling, a researcher starts by going where your participants are.
- Sampling frames can be real or hypothetical.
- Recruitment involves informing potential participants about your study and seeking their participation.
Glossary
- Exclusion criteria- characteristics that disqualify a person from being included in a sample
- Inclusion criteria- the characteristics a person must possess in order to be included in a sample
- Population- the cluster of people about whom a researcher is most interested
- Recruitment- the process by which the researcher informs potential participants about the study and attempts to get them to participate
- Sample- the group of people you successfully recruit from your sampling frame to participate in your study
- Sampling frame- a real or hypothetical list of people from which a researcher will draw her sample
6.2 Nonprobability sampling
Learning Objectives
- Define nonprobability sampling, and describe instances in which a researcher might choose a nonprobability sampling technique
- Describe the different types of nonprobability samples
Qualitative researchers typically make sampling choices that enable them to achieve a deep understanding of whatever phenomenon it is that they are studying. Sometimes quantitative researchers work with targeted or small samples. Qualitative research often employs a theoretical sampling strategy, where study sites, respondents, or cases are selected based on theoretical considerations such as whether they fit the phenomenon being studied (e.g., sustainable practices can only be studied in organizations that have implemented sustainable practices), whether they possess certain characteristics that make them uniquely suited for the study (e.g., a study of the drivers of firm innovations should include some firms that are high innovators and some that are low innovators, in order to draw contrast between these firms), and so forth. In this section, we’ll examine the techniques that these researchers typically employ when sampling as well as the various types of samples that they are most likely to use in their work.
Nonprobability sampling
Nonprobability sampling refers to sampling techniques for which a person’s likelihood of being selected for membership in the sample is unknown. Because we don’t know the likelihood of selection, with nonprobability samples we don’t know whether a sample is likely to represent a larger population. But that’s okay. Generalizing to a larger population is not the goal with nonprobability samples or qualitative research. That said, the fact that nonprobability samples do not represent a larger population does not mean that they are drawn arbitrarily or without any specific purpose in mind (that would mean committing one of the errors of informal inquiry discussed in Chapter 1). We’ll take a closer look at the process of selecting research elements when drawing a nonprobability sample. But first, let’s consider why a researcher might choose to use a nonprobability sample.
When are nonprobability samples ideal? One instance might be when we’re starting a big research project. For example, if we’re conducting survey research, we may want to administer a draft of our survey to a few people who seem to resemble the folks we’re interested in studying in order to help work out kinks in the survey. We might also use a nonprobability sample if we’re conducting a pilot study or some exploratory research. This can be a quick way to gather some initial data and help get some idea of the lay of the land before conducting a more extensive study. From these examples, we can see that nonprobability samples can be useful for setting up, framing, or beginning research, even quantitative research. But it isn’t just early stage research that relies on and benefits from nonprobability sampling techniques. Researchers also use nonprobability samples in advanced stage research projects. In this case, these projects are usually qualitative in nature, where the researcher’s goal is in-depth, idiographic understanding rather than more generalizable, nomothetic understanding.
Types of nonprobability samples
There are several types of nonprobability samples that researchers use. These include purposivesamples, snowball samples, quota samples, and convenience samples.
To draw a purposive sample, a researcher selects participants from a sampling frame because they have characteristics that the researcher desires. A researcher begins with specific characteristics in mind that she wishes to examine and then seeks out research participants who cover that full range of characteristics. For example, if you are studying mental health supports on your campus, you may want to be sure to include not only students, but mental health practitioners and student affairs administrators. You might also select students who currently use mental health supports, those who dropped out of supports, and those who are waiting to receive supports. The purposive part of purposive sampling comes from selecting specific participants on purpose because you already know they have characteristics—being an administrator, dropping out of mental health supports—that you need in your sample.
Note that these are different than inclusion criteria, which are more general requirements a person must possess to be a part of your sample. For example, one of the inclusion criteria for a study of your campus’ mental health supports might be that participants had to have visited the mental health center in the past year. That is different than purposive sampling. In purposive sampling, you know characteristics of individuals and recruit them because of those characteristics. For example, you might recruit Jane because she stopped seeking supports this month, JD because he has worked at the center for many years, and so forth.
Also, it’s important to recognize that purposive sampling requires you to have prior information about your participants before recruiting them because you need to know their perspectives or experiences before you know whether you want them in your sample. This is a common mistake that many students make. They may think they’re using purposive sampling because they’re recruiting people from the health center or something like that. That’s not purposive sampling. Purposive sampling is recruiting specific people because of the various characteristics and perspectives they bring to your sample. Imagine we were creating a focus group. A purposive sample might gather clinicians, patients, administrators, staff, and former patients together so they can talk as a group. Purposive sampling would seek out people that have each of those attributes.
Quota sampling is another nonprobability sampling strategy that takes purposive sampling one step further. When conducting quota sampling, a researcher identifies categories that are important to the study and for which there is likely to be some variation. Subgroups are created based on each category, and the researcher decides how many people to include from each subgroup and collects data from that number for each subgroup. Let’s consider a study of student satisfaction with on-campus housing. Perhaps there are two types of housing on your campus: apartments that include full kitchens and dorm rooms where residents do not cook for themselves and instead eat in a dorm cafeteria. As a researcher, you might wish to understand how satisfaction varies across these two types of housing arrangements. Perhaps you have the time and resources to interview 20 campus residents, so you decide to interview 10 from each housing type. It is possible as well that your review of literature on the topic suggests that campus housing experiences vary by gender. If that is that case, perhaps you’ll decide on four important subgroups: men who live in apartments, women who live in apartments, men who live in dorm rooms, and women who live in dorm rooms. Your quota sample would include five people from each of the four subgroups.
In 1936, up-and-coming pollster George Gallup made history when he successfully predicted the outcome of the presidential election using quota sampling methods. The leading polling entity at the time, The Literary Digest, predicted that Alfred Landon would beat Franklin Roosevelt in the presidential election by a landslide, but Gallup’s polling disagreed. Gallup successfully predicted Roosevelt’s win and subsequent elections based on quota samples, but in 1948, Gallup incorrectly predicted that Dewey would beat Truman in the US presidential election.[1] Among other problems, the fact that Gallup’s quota categories did not represent those who actually voted (Neuman, 2007) underscores the point that one should avoid attempting to make statistical generalizations from data collected using quota sampling methods. While quota sampling offers the strength of helping the researcher account for potentially relevant variation across study elements, it would be a mistake to think of this strategy as yielding statistically representative findings. For that, you need probability sampling, which we will discuss in the next section.
Researchers can also use snowball sampling techniques to identify study participants. In snowball sampling, a researcher identifies one or two people she’d like to include in her study and then relies on those initial participants to help identify additional study participants. Thus, the researcher’s sample builds and becomes larger as the study continues, much as a snowball builds and becomes larger as it rolls through the snow. Snowball sampling is an especially useful strategy when a researcher wishes to study a stigmatized group or behavior. For example, a researcher who wanted to study how people with genital herpes cope with their medical condition would be unlikely to find many participants by posting a call for interviewees in the newspaper or making an announcement about the study at some large social gathering. Instead, the researcher might know someone with the condition, interview that person, and ask the person to refer others they may know with the genital herpes to contact you to participate in the study. Having a previous participant vouch for the researcher may help new potential participants feel more comfortable about being included in the study.
Snowball sampling is sometimes referred to as chain referral sampling. One research participant refers another, and that person refers another, and that person refers another—thus a chain of potential participants is identified. In addition to using this sampling strategy for potentially stigmatized populations, it is also a useful strategy to use when the researcher’s group of interest is likely to be difficult to find, not only because of some stigma associated with the group, but also because the group may be relatively rare.
Steven Kogan and colleagues (2011) used a type sampling similar to snowball sampling called respondent-driven sampling (Heckathorn, 2012). They wished to study the sexual behaviors of non-college-bound African American young adults who lived in high-poverty rural areas. The researchers first relied on their own networks to identify study participants, but because members of the study’s target population were not easy to find, access to the networks of initial study participants was very important for identifying additional participants. Initial participants were given coupons to pass on to others they knew who qualified for the study. Participants were given an added incentive for referring eligible study participants; they received $50 for participating in the study and an additional $20 for each person they recruited who also participated in the study. Using this strategy, Kogan and colleagues succeeded in recruiting 292 study participants.
Finally, convenience sampling is another nonprobability sampling strategy that is employed by both qualitative and quantitative researchers. To draw a convenience sample, a researcher simply collects data from those people or other relevant elements to which she has most convenient access. This method, also sometimes referred to as availability sampling, is most useful in exploratory research or in student projects in which probability sampling is too costly or difficult. If you’ve ever been interviewed by a fellow student for a class project, you have likely been a part of a convenience sample. While convenience samples offer one major benefit—convenience—they do not offer the rigor needed to make conclusions about larger populations. That is the subject of our next section on probability sampling.
Sample type | Description |
Purposive | Researcher seeks out participants with specific characteristics. |
Snowball | Researcher relies on participant referrals to recruit new participants. |
Quota | Researcher selects cases from within several different subgroups. |
Convenience | Researcher gathers data from whatever cases happen to be convenient. |
Key Takeaways
- Nonprobability samples might be used when researchers are conducting qualitative (or idiographic) research, exploratory research, student projects, or pilot studies.
- There are several types of nonprobability samples including purposive samples, snowball samples, quota samples, and convenience samples.
Glossary
- Convenience sample- researcher gathers data from whatever cases happen to be convenient
- Nonprobability sampling- sampling techniques for which a person’s likelihood of being selected for membership in the sample is unknown
- Purposive sample- when a researcher seeks out participants with specific characteristics
- Quota sample- when a researcher selects cases from within several different subgroups
- Snowball sample- when a researcher relies on participant referrals to recruit new participants
6.3 Probability sampling
Learning Objectives
- Describe how probability sampling differs from nonprobability sampling
- Define generalizability, and describe how it is achieved in probability samples
- Identify the various types of probability samples, and describe why a researcher may use one type over another
Quantitative researchers are often interested in making generalizations about groups larger than their study samples — that is, they are seeking nomothetic causal explanations. While there are certainly instances when quantitative researchers rely on nonprobability samples (e.g., when doing exploratory research), quantitative researchers tend to rely on probability sampling techniques. The goals and techniques associated with probability samples differ from those of nonprobability samples. We’ll explore those unique goals and techniques in this section.
Probability sampling
Unlike nonprobability sampling, probability sampling refers to sampling techniques for which a person’s likelihood of being selected from the sampling frame is known. You might ask yourself why we should care about a potential participant’s likelihood of being selected for the researcher’s sample. The reason is that, in most cases, researchers who use probability sampling techniques are aiming to identify a representative sample from which to collect data. A representative sample is one that resembles the population from which it was drawn in all the ways that are important for the research being conducted. If, for example, you wish to be able to say something about differences between men and women at the end of your study, you better make sure that your sample doesn’t contain only women. That’s a bit of an oversimplification, but the point with representativeness is that if your population contains variations that are important to your study, your sample should contain the same sorts of variation.
Obtaining a representative sample is important in probability sampling because of generalizability. In fact, generalizability is perhaps the key feature that distinguishes probability samples from nonprobability samples. Generalizability refers to the idea that a study’s results will tell us something about a group larger than the sample from which the findings were generated. In order to achieve generalizability, a core principle of probability sampling is that all elements in the researcher’s sampling frame have an equal chance of being selected for inclusion in the study. In research, this is the principle of random selection. Researchers often use a computer’s random number generator to determine which elements from the sampling frame get recruited into the sample.
Using random selection does not mean that the sample will be perfect. No sample is perfect. The only way to come with a sample that perfectly reflects the population would be to include everyone in the population in your sample, which defeats the whole point of sampling! Generalizing from a sample to a population always contains some degree of error. This is referred to as sampling error, the difference between results from a sample and the actual values in the population.
Generalizability is a pretty easy concept to grasp. Imagine a professor who takes a sample of individuals in your class to see if the material is too hard or too easy. The professor, however, only sampled individuals whose grades were over 90% in the class. Would that be a representative sample of all students in the class? That would be a case of sampling error—a mismatch between the results of the sample and the true feelings of the overall class. In other words, the results of the professor’s study don’t generalize to the overall population of the class.
Taking this one step further, imagine your professor is conducting a study on binge drinking among college students. The professor uses undergraduates at your school as her sampling frame. Even if that professor were to use probability sampling, perhaps your school differs from other schools in important ways. There are schools that are “party schools” where binge drinking may be more socially accepted, “commuter schools” at which there is little nightlife, and so on. If your professor plans to generalize her results to all college students, she will have to make an argument that her sampling frame (undergraduates at your school) is representative of the population (all undergraduate college students).
Types of probability samples
There are a variety of types of probability samples that researchers may use. These include simple random samples, systematic random samples, stratified random samples, and cluster samples. Let’s build on the previous example. Imagine we were concerned with binge drinking and chose the target population of fraternity members. How might you go about getting a probability sample of fraternity members that is representative of the overall population?
Simple random sampling
Simple random samples are the most basic type of probability sample. A simple random sample requires a sampling frame than contains a list of each person in the sampling frame. Your school likely has a list of all of the fraternity members on campus, as Greek life is subject to university oversight. You could use this as your sampling frame. Using the university’s list, you would number each fraternity member, or element, sequentially and then randomly select the elements from which you will collect data.
True randomness is difficult to achieve, and it takes complex computational calculations to do so. Although you think you can select things at random, human-generated randomness is actually quite predictable. To truly randomly select elements, researchers must rely on computer-generated help. Many free websites have good pseudo-random number generators. A good example is the website Random.org, which contains a random number generator that can also randomize lists of participants. Sometimes, researchers use a table of numbers that have been generated randomly. There are several possible sources for obtaining a random number table. Some statistics and research methods textbooks offer such tables as appendices to the text.
Systematic random sampling
As you might have guessed, drawing a simple random sample can be quite tedious. Systematic random sampling techniques are somewhat less tedious but offer the benefits of a random sample. As with simple random samples, you must possess a list of everyone in your sampling frame. Once you’ve done that, to draw a systematic sample you’d simply select every kth element on your list. But what is k, and where on the list of population elements does one begin the selection process? k is your selection interval or the distance between the elements you select for inclusion in your study. To begin the selection process, you’ll need to figure out how many elements you wish to include in your sample. Let’s say you want to interview 25 fraternity members on your campus, and there are 100 men on campus who are members of fraternities. In this case, your selection interval, or k, is 4. To arrive at 4, simply divide the total number of population elements by your desired sample size. This process is represented in Figure 6.2.
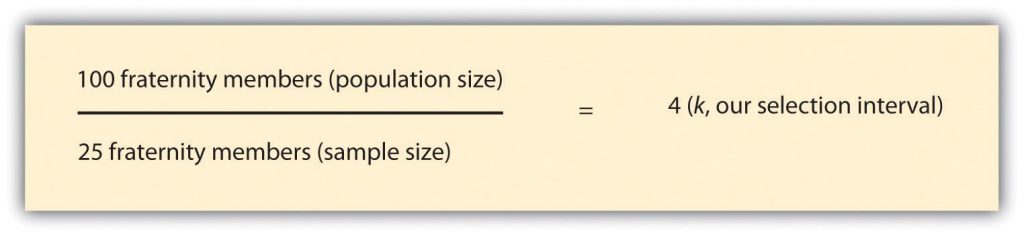
To determine where on your list of population elements to begin selecting the names of the 25 men you will interview, randomly select a number between 1 and k, and begin there. If we randomly select 3 as our starting point, we’d begin by selecting the third fraternity member on the list and then select every fourth member from there. This might be easier to understand if you can see it visually. Table 6.2 lists the names of our hypothetical 100 fraternity members on campus. You’ll see that the third name on the list has been selected for inclusion in our hypothetical study, as has every fourth name after that. A total of 25 names have been selected.
Number | Name | Include in study? | Number | Name | Include in study? | |
1 | Jacob | 51 | Blake | Yes | ||
2 | Ethan | 52 | Oliver | |||
3 | Michael | Yes | 53 | Cole | ||
4 | Jayden | 54 | Carlos | |||
5 | William | 55 | Jaden | Yes | ||
6 | Alexander | 56 | Jesus | |||
7 | Noah | Yes | 57 | Alex | ||
8 | Daniel | 58 | Aiden | |||
9 | Aiden | 59 | Eric | Yes | ||
10 | Anthony | 60 | Hayden | |||
11 | Joshua | Yes | 61 | Brian | ||
12 | Mason | 62 | Max | |||
13 | Christopher | 63 | Jaxon | Yes | ||
14 | Andrew | 64 | Brian | |||
15 | David | Yes | 65 | Mathew | ||
16 | Logan | 66 | Elijah | |||
17 | James | 67 | Joseph | Yes | ||
18 | Gabriel | 68 | Benjamin | |||
19 | Ryan | Yes | 69 | Samuel | ||
20 | Jackson | 70 | John | |||
21 | Nathan | 71 | Jonathan | Yes | ||
22 | Christian | 72 | Liam | |||
23 | Dylan | Yes | 73 | Landon | ||
24 | Caleb | 74 | Tyler | |||
25 | Lucas | 75 | Evan | Yes | ||
26 | Gavin | 76 | Nicholas | |||
27 | Isaac | Yes | 77 | Braden | ||
28 | Luke | 78 | Angel | |||
29 | Brandon | 79 | Jack | |||
30 | Isaiah | 80 | Jordan | |||
31 | Owen | Yes | 81 | Carter | ||
32 | Conner | 82 | Justin | |||
33 | Jose | 83 | Jeremiah | Yes | ||
34 | Julian | 84 | Robert | |||
35 | Aaron | Yes | 85 | Adrian | ||
36 | Wyatt | 86 | Kevin | |||
37 | Hunter | 87 | Cameron | Yes | ||
38 | Zachary | 88 | Thomas | |||
39 | Charles | Yes | 89 | Austin | ||
40 | Eli | 90 | Chase | |||
41 | Henry | 91 | Sebastian | Yes | ||
42 | Jason | 92 | Levi | |||
43 | Xavier | Yes | 93 | Ian | ||
44 | Colton | 94 | Dominic | |||
45 | Juan | 95 | Cooper | Yes | ||
46 | Josiah | 96 | Luis | |||
47 | Ayden | Yes | 97 | Carson | ||
48 | Adam | 98 | Nathaniel | |||
49 | Brody | 99 | Tristan | Yes | ||
50 | Diego | 100 | Parker | |||
There is one clear instance in which systematic sampling should not be employed. If your sampling frame has any pattern to it, you could inadvertently introduce bias into your sample by using a systemic sampling strategy. (Bias will be discussed in more depth in the next section.) This is sometimes referred to as the problem of periodicity. Periodicity refers to the tendency for a pattern to occur at regular intervals. Let’s say, for example, that you wanted to observe binge drinking on campus each day of the week. Perhaps you need to have your observations completed within 28 days and you wish to conduct four observations on randomly chosen days. Table 6.3 shows a list of the population elements for this example. To determine which days we’ll conduct our observations, we’ll need to determine our selection interval. As you’ll recall from the preceding paragraphs, to do so we must divide our population size, in this case 28 days, by our desired sample size, in this case 4 days. This formula leads us to a selection interval of 7. If we randomly select 2 as our starting point and select every seventh day after that, we’ll wind up with a total of 4 days on which to conduct our observations. You’ll see how that works out in the following table.
Day # | Day | Drinking | Observe? | Day # | Day | Drinking | Observe? | |
1 | Monday | Low | 15 | Monday | Low | |||
2 | Tuesday | Low | Yes | 16 | Tuesday | Low | Yes | |
3 | Wednesday | Low | 17 | Wednesday | Low | |||
4 | Thursday | High | 18 | Thursday | High | |||
5 | Friday | High | 19 | Friday | High | |||
6 | Saturday | High | 20 | Saturday | High | |||
7 | Sunday | Low | 21 | Sunday | Low | |||
8 | Monday | Low | 22 | Monday | Low | |||
9 | Tuesday | Low | Yes | 23 | Tuesday | Low | Yes | |
10 | Wednesday | Low | 24 | Wednesday | Low | |||
11 | Thursday | High | 25 | Thursday | High | |||
12 | Friday | High | 26 | Friday | High | |||
13 | Saturday | High | 27 | Saturday | High | |||
14 | Sunday | Low | 28 | Sunday | Low |
Do you notice any problems with our selection of observation days in Table 6.3? Apparently, we’ll only be observing on Tuesdays. Moreover, Tuesdays may not be an ideal day to observe binge drinking behavior because binge drinking may be more likely to happen over the weekend.
Stratified random sampling
Another type of random sampling that could be helpful in cases such as this is stratified random sampling. In stratified random sampling, a researcher divides the sampling frame into relevant subgroups and then draw a sample from each subgroup. In this example, we might wish to first divide our sampling frame into two lists: weekend and weekdays. Once we have our two lists, we can then apply either simple random or systematic sampling techniques to each subgroup.
Stratified sampling is a good technique to use when, as in our example, a subgroup of interest makes up a relatively small proportion of the overall sample. In our example of a study of binge drinking, we want to include weekdays and weekends in our sample, but because weekends make up less than a third of an entire week, there’s a chance that a simple random or systematic strategy would not yield sufficient weekend observation days. As you might imagine, stratified sampling is even more useful in cases where a subgroup makes up an even smaller proportion of the sampling frame—for example, if we want to be sure to include in our study students who are in year five of their undergraduate program but this subgroup makes up only a small percentage of the population of undergraduates. There’s a chance simple random or systematic sampling strategy might not yield any fifth-year students, but by using stratified sampling, we could ensure that our sample contained the proportion of fifth-year students that is reflective of the larger population.
In this case, class year (e.g., freshman, sophomore, junior, senior, and fifth-year and higher) is our strata, or the characteristic by which the sample is divided. In using stratified sampling, we are often concerned with how well our sample reflects the population. A sample with too many freshmen may skew our results in one direction because perhaps they binge drink more (or less) than students in other class years. Proportionate stratified random sampling allows us to make sure our sample has the same proportion of people from each class year as the overall population of the school. Disproportionate stratified random sampling allows us to over-sample smaller groups to ensure we have enough elements from the smaller group(s) for statistical analyses.
Cluster sampling
Up to this point in our discussion of probability samples, we’ve assumed that researchers will be able to access a list of population elements in order to create a sampling frame. This, as you might imagine, is not always the case. Let’s say, for example, that you wish to conduct a study of binge drinking across fraternity members at each undergraduate program in your state. Just imagine trying to create a list of every single fraternity member in the state. Even if you could find a way to generate such a list, attempting to do so might not be the most practical use of your time or resources. When this is the case, researchers turn to cluster sampling. Cluster sampling occurs when a researcher begins by randomly sampling groups (or clusters) of population elements and then selects elements from within those groups.
Let’s work through how we might use cluster sampling in our study of binge drinking. While creating a list of all fraternity members in your state would be next to impossible, you could easily create a list of all undergraduate colleges in your state. Thus, you could draw a random sample of undergraduate colleges (your cluster) and then draw another random sample of elements (in this case, fraternity members) from within the undergraduate college you initially selected. Cluster sampling works in stages. In this example, we sampled in two stages— (1) undergraduate colleges and (2) fraternity members at the undergraduate colleges we selected. However, we could add another stage if it made sense to do so. We could randomly select (1) undergraduate colleges (2) specific fraternities at each school and (3) individual fraternity members. As you might have guessed, sampling in multiple stages does introduce the possibility of greater error (each stage is subject to its own sampling error), but it is nevertheless highly efficien.
Jessica Holt and Wayne Gillespie (2008) used cluster sampling in their study of students’ experiences with violence in intimate relationships. Specifically, the researchers randomly selected 14 classes on their campus and then drew a random subsample of students from those classes. But you probably know from your experience with college classes that not all classes are the same size. So, if Holt and Gillespie had simply randomly selected 14 classes and then selected the same number of students from each class to complete their survey, then students in the smaller of those classes would have had a greater chance of being selected for the study than students in the larger classes. Keep in mind, with random sampling the goal is to make sure that each element has the same chance of being selected. When clusters are of different sizes, as in the example of sampling college classes, researchers often use a method called probability proportionate to size (PPS). This means that they take into account that their clusters are of different sizes. They do this by giving clusters different chances of being selected based on their size so that each element within those clusters winds up having an equal chance of being selected.
Comparing random sampling techniques
To summarize, probability samples are used to help a researcher make conclusions about larger groups. Probability samples require a sampling frame from which elements, usually human beings, can be selected at random from a list. The use of random selection reduces the error and bias present in the nonprobability sample types reviewed in the previous section, though some error will always remain. This strength is common to all probability sampling approaches summarized in Table 6.4.
Sample type | Description |
Simple random | Researcher randomly selects elements from sampling frame. |
Systematic random | Researcher selects every kth element from sampling frame. |
Stratified random | Researcher creates subgroups then randomly selects elements from each subgroup. |
Cluster | Researcher randomly selects clusters then randomly selects elements from selected clusters. |
In determining which probability sampling approach makes the most sense for your project, it helps to know more about your population. A simple random sample and systematic random sample are relatively similar to carry out. They both require a list of all elements in your sampling frame. Systematic random sampling is slightly easier in that it does not require you to use a random number generator for each element; instead it uses a sampling interval that is easy to calculate by hand.
The relative simplicity of both approaches is counter-weighted by their lack of sensitivity to characteristics in of your population. Stratified random samples help ensure that smaller subgroups are included in your sample, thus making the sample more representative of the overall population or allowing statistical analyses on subgroup differences possible. While these benefits are important, creating strata for this purpose requires knowing information about your population before beginning the sampling process. In our binge drinking example, we would need to know how many students are in each class year to make sure our sample contained the same proportions. We would need to know that, for example, fifth-year students make up 5% of the student population to make sure 5% of our sample is comprised of fifth-year students. If the true population parameters are unknown, stratified sampling becomes significantly more challenging.
Common to each of the previous probability sampling approaches is the necessity of using a real list of all elements in your sampling frame. Cluster sampling is different. It allows a researcher to perform probability sampling in cases for which a list of elements is not available or pragmatic to create. Cluster sampling is also useful for making claims about a larger population, in our example, all fraternity members within a state. However, because sampling occurs at multiple stages in the process, in our example at the university and student level, sampling error increases. For many researchers, this weakness is outweighed by the benefits of cluster sampling.
Key Takeaways
- In probability sampling, the aim is to identify a sample that resembles the population from which it was drawn.
- There are several types of probability samples including simple random samples, systematic samples, stratified samples, and cluster samples.
- Probability samples usually require a real list of elements in your sampling frame, though cluster sampling can be conducted without one.
Glossary
- Cluster sampling- a sampling approach that begins by sampling groups (or clusters) of population elements and then selects elements from within those groups
- Disproportionate stratified random sampling-stratified random sampling where the proportion of elements from each group is not proportionate to that in the population (usually used to oversample small groups).
- Generalizability – the idea that a study’s results will tell us something about a group larger than the sample from which the findings were generated
- Periodicity- the tendency for a pattern to occur at regular intervals
- Probability proportionate to size- in cluster sampling, giving clusters different chances of being selected based on their size so that each element within those clusters has an equal chance of being selected
- Probability sampling- sampling approaches for which a person’s (or element’s) likelihood of being selected from the sampling frame is known
- Proportionate stratified random sampling-stratified random sampling where the proportion of elements from each group is proportionate to that in the population
- Random selection- using a randomly generated numbers to determine who from the sampling frame gets recruited into the sample
- Representative sample- a sample that resembles the population from which it was drawn in all the ways that are important for the research being conducted
- Sampling error- a statistical calculation of the difference between results from a sample and the actual parameters of a population
- Simple random sampling- selecting elements from a list using randomly generated numbers
- Strata- the characteristic by which the sample is divided
- Stratified random sampling- dividing the study population into relevant subgroups and then draw a sample from each subgroup
- Systematic random sampling- selecting every kth element from a list
6.4 Critical thinking about samples
Learning Objectives
- Identify three questions you should ask about samples when reading research results
- Describe how bias impacts sampling
We read and hear about research results so often that we might sometimes overlook the need to ask important questions about where the research participants came from and how they are identified for inclusion. It is easy to focus only on findings when we’re busy and when the really interesting stuff is in a study’s conclusions, not its procedures. But now that you have some familiarity with the variety of procedures for selecting study participants, you are equipped to ask some very important questions about the findings you read and to be a more responsible consumer of research.
Who was sampled, how, and for what purpose?
Have you ever been a participant in someone’s research? If you have ever taken an introductory psychology or sociology class at a large university, that’s probably a silly question to ask. Social science researchers on college campuses have a luxury that researchers elsewhere may not share—they have access to a whole bunch of (presumably) willing and able human guinea pigs. But that luxury comes at a cost—sample representativeness. One study of top academic journals in psychology found that over two-thirds (68%) of participants in studies published by those journals were based on samples drawn in the United States (Arnett, 2008). Further, the study found that two-thirds of the work that derived from U.S. samples published in the Journal of Personality and Social Psychology was based on samples made up entirely of American undergraduates taking psychology courses.
These findings certainly raise the question: What do we actually learn from social scientific studies and about whom do we learn it? That is exactly the concern raised by Joseph Henrich and colleagues (Henrich, Heine, & Norenzayan, 2010), authors of the article “The Weirdest People in the World?” In their piece, Henrich and colleagues point out that behavioral scientists very commonly make sweeping claims about human nature based on samples drawn only from WEIRD (Western, Educated, Industrialized, Rich, and Democratic) societies, and often based on even narrower samples, as is the case with many studies relying on samples drawn from college classrooms. As it turns out, many robust findings about the nature of human behavior when it comes to fairness, cooperation, visual perception, trust, and other behaviors are based on studies that excluded participants from outside the United States and sometimes excluded anyone outside the college classroom (Begley, 2010). This certainly raises questions about what we really know about human behavior as opposed to U.S. resident or U.S. undergraduate behavior. Of course, not all research findings are based on samples of WEIRD folks. But even then, it would behoove us to pay attention to the population on which studies are based and the claims that are being made about to whom those studies apply.
In the preceding discussion, the concern is with researchers making claims about populations other than those from which their samples were drawn. A related, but slightly different, potential concern is selection bias. Selection bias occurs when the elements selected for inclusion in a study do not represent the larger population from which they were drawn. For example, if you were to sample people walking into the social work building on campus during each weekday, your sample would include too many social work majors and not enough non-social work majors. Furthermore, you would completely exclude students whose classes are at night. Bias may be introduced by the sampling method used or due to conscious or unconscious bias introduced by the researcher (Rubin & Babbie, 2017). A researcher might select people who “look like good research participants,” in the process transferring their unconscious biases to their sample.
Another thing to keep in mind is that just because a sample may be representative in all respects that a researcher thinks are relevant, there may be aspects that are relevant that didn’t occur to the researcher when she was drawing her sample. You might not think that a person’s phone would have much to do with their voting preferences, for example. But had pollsters making predictions about the results of the 2008 presidential election not been careful to include both cell phone-only and landline households in their surveys, it is possible that their predictions would have underestimated Barack Obama’s lead over John McCain because Obama was much more popular among cell-only users than McCain (Keeter, Dimock, & Christian, 2008).
So how do we know when we can count on results that are being reported to us? While there might not be any magic or always-true rules we can apply, there are a couple of things we can keep in mind as we read the claims researchers make about their findings.
First, remember that sample quality is determined by the sample actually obtained, not by the sampling method itself. A researcher may set out to administer a survey to a representative sample by correctly employing a random selection technique, but if only a handful of the people sampled actually respond to the survey, the researcher will have to be very careful about the claims she can make about her survey findings.
Another thing to keep in mind, as demonstrated by the preceding discussion, is that researchers may be drawn to talking about implications of their findings as though they apply to some group other than the population actually sampled. Though this tendency is usually quite innocent and does not come from a place of malice, it is all too tempting a way to talk about findings; as consumers of those findings, it is our responsibility to be attentive to this sort of (likely unintentional) bait and switch.
Finally, keep in mind that a sample that allows for comparisons of theoretically important concepts or variables is certainly better than one that does not allow for such comparisons. In a study based on a nonrepresentative sample, for example, we can learn about the strength of our social theories by comparing relevant aspects of social processes. We talked about this as theory-testing in Chapter 4.
At their core, questions about sample quality should address who has been sampled, how they were sampled, and for what purpose they were sampled. Being able to answer those questions will help you better understand, and more responsibly read, research results.
Key Takeaways
- Sometimes researchers may make claims about populations other than those from whom their samples were drawn; other times they may make claims about a population based on a sample that is not representative. As consumers of research, we should be attentive to both possibilities.
- A researcher’s findings need not be generalizable to be valuable; samples that allow for comparisons of theoretically important concepts or variables may yield findings that contribute to our social theories and our understandings of social processes.
Glossary
- Selection bias- when the elements selected for inclusion in a study do not represent the larger population from which they were drawn due to sampling method or thought processes of the researcher