This chapter is mainly focused on quantitative research methods, as the level of specificity required to begin quantitative research is far greater than that of qualitative research. In quantitative research, you must specify how you define and plan to measure each concept before you can interact with your participants. In qualitative research, definitions emerge from how participants respond to your questions. Because your participants are the experts, qualitative research does not begin with defining concepts at the level of specificity and clarity required for quantitative research. For this reason, we will focus mostly on quantitative measurement and conceptualization in this chapter, addressing qualitative research later in the textbook.
Chapter Outline
- 5.1 Measurement
- 5.2 Conceptualization
- 5.3 Levels of measurement
- 5.4 Operationalization
- 5.5 Measurement quality
- 5.6 Challenges in quantitative measurement
Content Advisory
This chapter includes information or discusses measuring depression and loneliness among residents of an assisted living facility.
5.1 Measurement
Learning Objectives
- Define measurement
- Describe Kaplan’s three categories of the things that social scientists measure
Measurement is important. Recognizing that fact, and respecting it, will be of great benefit to you—both in research methods and in other areas of life as well. Measurement is critical to successfully pulling off a social scientific research project. In social science, when we use the term measurement we mean the process by which we describe and ascribe meaning to the key facts, concepts, or other phenomena that we are investigating. At its core, measurement is about defining one’s terms in as clear and precise a way as possible. Of course, measurement in social science isn’t quite as simple as using a measuring cup or spoon, but there are some basic tenants on which most social scientists agree when it comes to measurement. We’ll explore those, as well as some of the ways that measurement might vary depending on your unique approach to the study of your topic.
What do social scientists measure?
The question of what social scientists measure can be answered by asking yourself what social scientists study. Think about the topics you’ve learned about in other social work classes you’ve taken or the topics you’ve considered investigating yourself. Let’s consider Melissa Milkie and Catharine Warner’s study (2011) of first graders’ mental health. In order to conduct that study, Milkie and Warner needed to have some idea about how they were going to measure mental health. What does mental health mean, exactly? And how do we know when we’re observing someone whose mental health is good and when we see someone whose mental health is compromised? Understanding how measurement works in research methods helps us answer these sorts of questions.
As you might have guessed, social scientists will measure just about anything that they have an interest in investigating. For example, those who are interested in learning something about the correlation between social class and levels of happiness must develop some way to measure both social class and happiness. Those who wish to understand how well immigrants cope in their new locations must measure immigrant status and coping. Those who wish to understand how a person’s gender shapes their workplace experiences must measure gender and workplace experiences. You get the idea. Social scientists can and do measure just about anything you can imagine observing or wanting to study. Of course, some things are easier to observe or measure than others.
In 1964, philosopher Abraham Kaplan (1964) wrote TheConduct of Inquiry, which has since become a classic work in research methodology (Babbie, 2010). In his text, Kaplan describes different categories of things that behavioral scientists observe. One of those categories, which Kaplan called “observational terms,” is probably the simplest to measure in social science. Observational terms are the sorts of things that we can see with the naked eye simply by looking at them. They are terms that “lend themselves to easy and confident verification” (Kaplan, 1964, p. 54). If, for example, we wanted to know how the conditions of playgrounds differ across different neighborhoods, we could directly observe the variety, amount, and condition of equipment at various playgrounds.
Indirect observables, on the other hand, are less straightforward to assess. They are “terms whose application calls for relatively more subtle, complex, or indirect observations, in which inferences play an acknowledged part. Such inferences concern presumed connections, usually causal, between what is directly observed and what the term signifies” (Kaplan, 1964, p. 55). If we conducted a study for which we wished to know a person’s income, we’d probably have to ask them their income, perhaps in an interview or a survey. Thus, we have observed income, even if it has only been observed indirectly. Birthplace might be another indirect observable. We can ask study participants where they were born, but chances are good we won’t have directly observed any of those people being born in the locations they report.
Sometimes the measures that we are interested in are more complex and more abstract than observational terms or indirect observables. Think about some of the concepts you’ve learned about in other social work classes—for example, ethnocentrism. What is ethnocentrism? Well, from completing an introduction to social work class you might know that it has something to do with the way a person judges another’s culture. But how would you measure it? Here’s another construct: bureaucracy. We know this term has something to do with organizations and how they operate, but measuring such a construct is trickier than measuring, say, a person’s income. In both cases, ethnocentrism and bureaucracy, these theoretical notions represent ideas whose meaning we have come to agree on. Though we may not be able to observe these abstractions directly, we can observe the things that they are made up of.
Kaplan referred to these more abstract things that behavioral scientists measure as constructs. Constructs are “not observational either directly or indirectly” (Kaplan, 1964, p. 55), but they can be defined based on observables. For example, the construct of bureaucracy could be measured by counting the number of supervisors that need to approve routine spending by public administrators. The greater the number of administrators that must sign off on routine matters, the greater the degree of bureaucracy. Similarly, we might be able to ask a person the degree to which they trust people from different cultures around the world and then assess the ethnocentrism inherent in their answers. We can measure constructs like bureaucracy and ethnocentrism by defining them in terms of what we can observe.
Thus far, we have learned that social scientists measure what Kaplan called observational terms, indirect observables, and constructs. These terms refer to the different sorts of things that social scientists may be interested in measuring. But how do social scientists measure these things? That is the next question we’ll tackle.
How do social scientists measure?
Measurement in social science is a process. It occurs at multiple stages of a research project: in the planning stages, in the data collection stage, and sometimes even in the analysis stage. Recall that previously we defined measurement as the process by which we describe and ascribe meaning to the key facts, concepts, or other phenomena that we are investigating. Once we’ve identified a research question, we begin to think about what some of the key ideas are that we hope to learn from our project. In describing those key ideas, we begin the measurement process.
Let’s say that our research question is the following: How do new college students cope with the adjustment to college? In order to answer this question, we’ll need some idea about what coping means. We may come up with an idea about what coping means early in the research process, as we begin to think about what to look for (or observe) in our data-collection phase. Once we’ve collected data on coping, we also have to decide how to report on the topic. Perhaps, for example, there are different types or dimensions of coping, some of which lead to more successful adjustment than others. However we decide to proceed, and whatever we decide to report, the point is that measurement is important at each of these phases.
As the preceding example demonstrates, measurement is a process in part because it occurs at multiple stages of conducting research. We could also think of measurement as a process because it involves multiple stages. From identifying your key terms to defining them to figuring out how to observe them and how to know if your observations are any good, there are multiple steps involved in the measurement process. An additional step in the measurement process involves deciding what elements your measures contain. A measure’s elements might be very straightforward and clear, particularly if they are directly observable. Other measures are more complex and might require the researcher to account for different themes or types. These sorts of complexities require paying careful attention to a concept’s level of measurement and its dimensions. We’ll explore these complexities in greater depth at the end of this chapter, but first let’s look more closely at the early steps involved in the measurement process, starting with conceptualization.
Key Takeaways
- Measurement is the process by which we describe and ascribe meaning to the key facts, concepts, or other phenomena that we are investigating.
- Kaplan identified three categories of things that social scientists measure including observational terms, indirect observables, and constructs.
- Measurement occurs at all stages of research.
Glossary
- Constructs- are not observable but can be defined based on observable characteristics
- Indirect observables- things that require indirect observation and inference to measure
- Measurement- the process by which researchers describe and ascribe meaning to the key facts, concepts, or other phenomena they are investigating
- Observational terms- things that we can see with the naked eye simply by looking at them
5.2 Conceptualization
Learning Objectives
- Define concept
- Identify why defining our concepts is important
- Describe how conceptualization works in quantitative and qualitative research
- Define dimensions in terms of social scientific measurement
- Apply reification to conceptualization
In this section, we’ll take a look at one of the first steps in the measurement process, which is conceptualization. This has to do with defining our terms as clearly as possible and also not taking ourselves too seriously in the process. Our definitions mean only what we say they mean—nothing more and nothing less. Let’s talk first about how to define our terms, and then we’ll examine not taking ourselves (or our terms, rather) too seriously.
Concepts and conceptualization
So far, the word concept has come up quite a bit, and it would behoove us to make sure we have a shared understanding of that term. A concept is the notion or image that we conjure up when we think of some cluster of related observations or ideas. For example, masculinity is a concept. What do you think of when you hear that word? Presumably, you imagine some set of behaviors and perhaps even a particular style of self-presentation. Of course, we can’t necessarily assume that everyone conjures up the same set of ideas or images when they hear the word masculinity. In fact, there are many possible ways to define the term. And while some definitions may be more common or have more support than others, there isn’t one true, always-correct-in-all-settings definition. What counts as masculine may shift over time, from culture to culture, and even from individual to individual (Kimmel, 2008). This is why defining our concepts is so important.
You might be asking yourself why you should bother defining a term for which there is no single, correct definition. Believe it or not, this is true for any concept you might measure in a research study—there is never a single, always-correct definition. When we conduct empirical research, our terms mean only what we say they mean. There’s a New Yorker cartoon that aptly represents this idea. It depicts a young George Washington holding an axe and standing near a freshly chopped cherry tree. Young George is looking up at a frowning adult who is standing over him, arms crossed. The caption depicts George explaining, “It all depends on how you define ‘chop.’” Young George Washington gets the idea—whether he actually chopped down the cherry tree depends on whether we have a shared understanding of the term chop.
Without a shared understanding of this term, our understandings of what George has just done may differ. Likewise, without understanding how a researcher has defined her key concepts, it would be nearly impossible to understand the meaning of that researcher’s findings and conclusions. Thus, any decision we make based on findings from empirical research should be made based on full knowledge not only of how the research was designed, but also of how its concepts were defined and measured.
So, how do we define our concepts? This is part of the process of measurement, and this portion of the process is called conceptualization. The answer depends on how we plan to approach our research. We will begin with quantitative conceptualization and then discuss qualitative conceptualization.
In quantitative research, conceptualization involves writing out clear, concise definitions for our key concepts. Sticking with the previously mentioned example of masculinity, think about what comes to mind when you read that term. How do you know masculinity when you see it? Does it have something to do with men? With social norms? If so, perhaps we could define masculinity as the social norms that men are expected to follow. That seems like a reasonable start, and at this early stage of conceptualization, brainstorming about the images conjured up by concepts and playing around with possible definitions is appropriate. However, this is just the first step.
It would make sense as well to consult other previous research and theory to understand if other scholars have already defined the concepts we’re interested in. This doesn’t necessarily mean we must use their definitions, but understanding how concepts have been defined in the past will give us an idea about how our conceptualizations compare with the predominant ones out there. Understanding prior definitions of our key concepts will also help us decide whether we plan to challenge those conceptualizations or rely on them for our own work. Finally, working on conceptualization is likely to help in the process of refining your research question to one that is specific and clear in what it asks.
If we turn to the literature on masculinity, we will surely come across work by Michael Kimmel, one of the preeminent masculinity scholars in the United States. After consulting Kimmel’s prior work (2000; 2008), we might tweak our initial definition of masculinity just a bit. Rather than defining masculinity as “the social norms that men are expected to follow,” perhaps instead we’ll define it as “the social roles, behaviors, and meanings prescribed for men in any given society at any one time” (Kimmel & Aronson, 2004, p. 503). Our revised definition is both more precise and more complex. Rather than simply addressing one aspect of men’s lives (norms), our new definition addresses three aspects: roles, behaviors, and meanings. It also implies that roles, behaviors, and meanings may vary across societies and over time. To be clear, we’ll also have to specify the particular society and time period we’re investigating as we conceptualize masculinity.
As you can see, conceptualization isn’t quite as simple as merely applying any random definition that we come up with to a term. Sure, it may involve some initial brainstorming, but conceptualization goes beyond that. Once we’ve brainstormed a bit about the images a particular word conjures up for us, we should also consult prior work to understand how others define the term in question. And after we’ve identified a clear definition that we’re happy with, we should make sure that every term used in our definition will make sense to others. Are there terms used within our definition that also need to be defined? If so, our conceptualization is not yet complete. And there is yet another aspect of conceptualization to consider—concept dimensions. We’ll consider that aspect along with an additional word of caution about conceptualization in the next subsection.
Conceptualization in qualitative research
Conceptualization in qualitative research proceeds a bit differently than in quantitative research. Because qualitative researchers are interested in the understandings and experiences of their participants, it is less important for the researcher to find one fixed definition for a concept before starting to interview or interact with participants. The researcher’s job is to accurately and completely represent how their participants understand a concept, not to test their own definition of that concept.
If you were conducting qualitative research on masculinity, you would likely consult previous literature like Kimmel’s work mentioned above. From your literature review, you may come up with a working definition for the terms you plan to use in your study, which can change over the course of the investigation. However, the definition that matters is the definition that your participants share during data collection. A working definition is merely a place to start, and researchers should take care not to think it is the only or best definition out there.
In qualitative inquiry, your participants are the experts (sound familiar, social workers?) on the concepts that arise during the research study. Your job as the researcher is to accurately and reliably collect and interpret their understanding of the concepts they describe while answering your questions. Conceptualization of qualitative concepts is likely to change over the course of qualitative inquiry, as you learn more information from your participants. Indeed, getting participants to comment on, extend, or challenge the definitions and understandings of other participants is a hallmark of qualitative research. This is the opposite of quantitative research, in which definitions must be completely set in stone before the inquiry can begin.
A word of caution about conceptualization
Whether you have chosen qualitative or quantitative methods, you should have a clear definition for the term masculinity and make sure that the terms we use in our definition are equally clear—and then we’re done, right? Not so fast. If you’ve ever met more than one man in your life, you’ve probably noticed that they are not all exactly the same, even if they live in the same society and at the same historical time period. This could mean there are dimensions of masculinity. In terms of social scientific measurement, concepts can be said to have multiple dimensions when there are multiple elements that make up a single concept. With respect to the term masculinity, dimensions could be regional (is masculinity defined differently in different regions of the same country?), age-based (is masculinity defined differently for men of different ages?), or perhaps power-based (does masculinity differ based on membership to privileged groups?). In any of these cases, the concept of masculinity would be considered to have multiple dimensions. While it isn’t necessarily required to spell out every possible dimension of the concepts you wish to measure, it may be important to do so depending on the goals of your research. The point here is to be aware that some concepts have dimensions and to think about whether and when dimensions may be relevant to the concepts you intend to investigate.
Before we move on to the additional steps involved in the measurement process, it would be wise to remind ourselves not to take our definitions too seriously. Conceptualization must be open to revisions, even radical revisions, as scientific knowledge progresses. Although that we should consult prior scholarly definitions of our concepts, it would be wrong to assume that just because prior definitions exist that they are more real than the definitions we create (or, likewise, that our own made-up definitions are any more real than any other definition). It would also be wrong to assume that just because definitions exist for some concept that the concept itself exists beyond some abstract idea in our heads. This idea, assuming that our abstract concepts exist in some concrete, tangible way, is known as reification.
To better understand reification, take a moment to think about the concept of social structure. This concept is central to critical thinking. When social scientists talk about social structure, they are talking about an abstract concept. Social structures shape our ways of being in the world and of interacting with one another, but they do not exist in any concrete or tangible way. A social structure isn’t the same thing as other sorts of structures, such as buildings or bridges. Sure, both types of structures are important to how we live our everyday lives, but one we can touch, and the other is just an idea that shapes our way of living.
Here’s another way of thinking about reification: Think about the term family. If you were interested in studying this concept, we’ve learned that it would be good to consult prior theory and research to understand how the term has been conceptualized by others. But we should also question past conceptualizations. Think, for example, about how different the definition of family was 50 years ago. Because researchers from that time period conceptualized family using now outdated social norms, social scientists from 50 years ago created research projects based on what we consider now to be a very limited and problematic notion of what family means. Their definitions of family were as real to them as our definitions are to us today. If researchers never challenged the definitions of terms like family, our scientific knowledge would be filled with the prejudices and blind spots from years ago. It makes sense to come to some social agreement about what various concepts mean. Without that agreement, it would be difficult to navigate through everyday living. But at the same time, we should not forget that we have assigned those definitions, they are imperfect and subject to change as a result of critical inquiry.
Key Takeaways
- Conceptualization is a process that involves coming up with clear, concise definitions.
- Conceptualization in quantitative research comes from the researcher’s ideas or the literature.
- Qualitative researchers conceptualize by creating working definitions which will be revised based on what participants say.
- Some concepts have multiple elements or dimensions.
- Researchers should acknowledge the limitations of their definitions for concepts.
Glossary
- Concept- notion or image that we conjure up when we think of some cluster of related observations or ideas
- Conceptualization- writing out clear, concise definitions for our key concepts, particularly in quantitative research
- Multi-dimensional concepts- concepts that are comprised of multiple elements
- Reification- assuming that abstract concepts exist in some concrete, tangible way
5.3 Levels of measurement
Learning Objectives
- Define and provide examples for the four levels of measurement
Now that we have figured out how to define, or conceptualize, our terms we’ll need to think about operationalizing them. Operationalization is the process by which researchers conducting quantitative research spell out precisely how a concept will be measured. It involves identifying the specific research procedures we will use to gather data about our concepts. This of course requires that we know what research method(s) we will employ to learn about our concepts, and we’ll examine specific research methods later on in the text. For now, let’s take a broad look at how operationalization works. We can then revisit how this process works when we examine specific methods of data collection in later chapters. Remember, operationalization is only a process in quantitative research. Measurement in qualitative research will be discussed at the end of this section.
Levels of measurement
When social scientists measure concepts, they sometimes use the language of variables and attributes (also called values). A variable refers to a phenomenon that can vary. It can be thought of as a grouping of several characteristics. For example, hair color could be a variable because it has varying characteristics. Attributes are the characteristics that make up a variable. For example, the variable hair color would contain attributes like blonde, brown, black, red, gray, etc.
A variable’s attributes determine its level of measurement. There are four possible levels of measurement: nominal, ordinal, interval, and ratio. The first two levels of measurement are categorical, meaning their attributes are categories rather than numbers. The latter two levels of measurement are continuous, meaning their attributes are numbers, not categories.
Nominal level of measurement
Hair color is an example of a nominal level of measurement. Nominal measures are categorical, and those categories cannot be mathematically ranked. There is no ranking order between hair colors. They are simply different. That is what constitutes a nominal level of measurement. Gender and race are also measured at the nominal level.
When using nominal level of measurement in research, it is very important to assign the attributes of potential answers very precisely. The attributes need to be exhaustive and mutually exclusive. Let’s think about the attributes contained in the variable hair color. Black, brown, blonde, and red are common colors. But, if we listed only these attributes, people with gray hair wouldn’t fit anywhere. That means our attributes were not exhaustive. Exhaustiveness means that all possible attributes are listed. We may have to list a lot of colors before we can meet the criteria of exhaustiveness. Clearly, there is a point at which trying to achieve exhaustiveness can get to be too much. If a person insists that their hair color is light burnt sienna, it is not your responsibility to list that as an option. Rather, that person could reasonably be described as brown-haired. Perhaps listing a category for other color would suffice to make our list of colors exhaustive.
What about a person who has multiple hair colors at the same time, such as red and black? They would fall into multiple attributes. This violates the rule of mutual exclusivity, in which a person cannot fall into two different attributes. Instead of listing all of the possible combinations of colors, perhaps you might include a list of attributes like all black, all brown, all blonde, all red, multi-color, other to include people with more than one hair color, but keep everyone in only one category.
The discussion of hair color elides an important point with measurement—reification. You should remember reification from our previous discussion in this chapter. For many years, the attributes for gender were male and female. Now, our understanding of gender has evolved to encompass more attributes including transgender, non-binary, or genderqueer. We shouldn’t confuse our labeling of attributes or measuring of a variable with the objective truth “out there.” Another example could be children of parents from different races were often classified as one race or another in the past, even if they identified with both cultures equally. The option for bi-racial or multi-racial on a survey not only more accurately reflects the racial diversity in the real world but validates and acknowledges people who identify in that manner.
Ordinal level of measurement
Unlike nominal-level measures, attributes at the ordinal level can be rank ordered. For example, someone’s degree of satisfaction in their romantic relationship can be ordered by rank. That is, you could say you are not at all satisfied, a little satisfied, moderately satisfied, or highly satisfied. Note that even though these have a rank order to them (not at all satisfied is certainly worse than highly satisfied), we cannot calculate a mathematical distance between those attributes. We can simply say that one attribute of an ordinal-level variable is more or less than another attribute.
This can get a little confusing when using Likertscales. If you have ever taken a customer satisfaction survey or completed a course evaluation for school, you are familiar with Likert scales. “On a scale of 1-5, with one being the lowest and 5 being the highest, how likely are you to recommend our company to other people?” Sound familiar? Likert scales use numbers but only as a shorthand to indicate what attribute (highly likely, somewhat likely, etc.) the person feels describes them best. You wouldn’t say you are “2” more likely to recommend the company. But you could say you are not very likely to recommend the company.
Ordinal-level attributes must also be exhaustive and mutually exclusive, as with nominal-level variables.
Interval level of measurement
At the interval level, the distance between attributes is known to be equal. Interval measures are also continuous, meaning their attributes are numbers, rather than categories. IQ scores are interval level, as are temperatures. Interval-level variables are not particularly common in social science research, but their defining characteristic is that we can say how much more or less one attribute differs from another. We cannot, however, say with certainty what the ratio of one attribute is in comparison to another. For example, it would not make sense to say that 50 degrees is half as hot as 100 degrees. But we can say it is 50 degrees cooler than 100. At the interval level, attributes must also be exhaustive and mutually exclusive.
Ratio level of measurement
Finally, at the ratio level, attributes can be rank ordered, the distance between attributes is equal, and attributes have a true zero point. Thus, with these variables, we can say what the ratio of one attribute is in comparison to another. Examples of ratio-level variables include age and years of education. We know, for example, that a person who is 12 years old is twice as old as someone who is 6 years old. Just like all other levels of measurement, at the ratio level, attributes must be mutually exclusive and exhaustive.
The differences between each level of measurement are visualized in Table 5.1.
Nominal | Ordinal | Interval | Ratio | |
Exhaustive | X | X | X | X |
Mutually exclusive | X | X | X | X |
Rank-ordered | X | X | X | |
Equal distance between attributes | X | X | ||
Can compare ratios of the values (e.g., twice as large) | X | |||
True zero point | X |
Key Takeaways
- In social science, our variables can be one of four different levels of measurement: nominal, ordinal, interval, or ratio.
Glossary
- Categorical measures- a measure with attributes that are categories
- Continuous measures- a measures with attributes that are numbers
- Exhaustiveness- all possible attributes are listed
- Interval level- a level of measurement that is continuous, can be rank ordered, is exhaustive and mutually exclusive, and for which the distance between attributes is known to be equal
- Likert scales- ordinal measures that use numbers as a shorthand (e.g., 1=highly likely, 2=somewhat likely, etc.) to indicate what attribute the person feels describes them best
- Mutual exclusivity- a person cannot identify with two different attributes simultaneously
- Nominal- level of measurement that is categorical and those categories cannot be mathematically ranked, though they are exhaustive and mutually exclusive
- Ordinal- level of measurement that is categorical, those categories can be rank ordered, and they are exhaustive and mutually exclusive
- Ratio level- level of measurement in which attributes are mutually exclusive and exhaustive, attributes can be rank ordered, the distance between attributes is equal, and attributes have a true zero point
- Variable- refers to a grouping of several characteristics
5.4 Operationalization
Learning Objectives
- Define and give an example of indicators for a variable
- Identify the three components of an operational definition
- Describe the purpose of multi-dimensional measures such as indexes, scales, and typologies and why they are used
Now that we have figured out how to define, or conceptualize, our terms we’ll need to think about operationalizing them. Operationalization is the process by which researchers conducting quantitative research spell out precisely how a concept will be measured. It involves identifying the specific research procedures we will use to gather data about our concepts. This of course requires that we know what research method(s) we will employ to learn about our concepts, and we’ll examine specific research methods later on in the text. For now, let’s take a broad look at how operationalization works. We can then revisit how this process works when we examine specific methods of data collection in later chapters. Remember, operationalization is only a process in quantitative research. Measurement in qualitative research will be discussed at the end of this section.
Operationalization works by identifying specific indicators that will be taken to represent the ideas we are interested in studying. If, for example, we are interested in studying masculinity, indicators for that concept might include some of the social roles prescribed to men in society such as breadwinning or fatherhood. Being a breadwinner or a father might therefore be considered indicators of a person’s masculinity. The extent to which a man fulfills either, or both, of these roles might be understood as clues (or indicators) about the extent to which he is viewed as masculine.
Let’s look at another example of indicators. Each day, Gallup researchers poll 1,000 randomly selected Americans to ask them about their well-being. To measure well-being, Gallup asks these people to respond to questions covering six broad areas: physical health, emotional health, work environment, life evaluation, healthy behaviors, and access to basic necessities. Gallup uses these six factors as indicators of the concept that they are really interested in, which is well-being.
Identifying indicators can be even simpler than the examples described thus far. What are the possible indicators of the concept of gender? Most of us would probably agree that “man” and “woman” are both reasonable indicators of gender, but you may want to include other options for people who identify as non-binary or other genders. Political party is another relatively easy concept for which to identify indicators. In the United States, likely indicators include Democrat and Republican and, depending on your research interest, you may include additional indicators such as Independent, Green, or Libertarian as well. Age and birthplace are additional examples of concepts for which identifying indicators is a relatively simple process. What concepts are of interest to you, and what are the possible indicators of those concepts?
We have now considered a few examples of concepts and their indicators, but it is important we don’t make the process of coming up with indicators too arbitrary or casual. One way to avoid taking an overly casual approach in identifying indicators, as described previously, is to turn to prior theoretical and empirical work in your area. Theories will point you in the direction of relevant concepts and possible indicators; empirical work will give you some very specific examples of how the important concepts in an area have been measured in the past and what sorts of indicators have been used. Often, it makes sense to use the same indicators as researchers who have come before you. On the other hand, perhaps you notice some possible weaknesses in measures that have been used in the past that your own methodological approach will enable you to overcome.
Speaking of your methodological approach, another very important thing to think about when deciding on indicators and how you will measure your key concepts is the strategy you will use for data collection. A survey implies one way of measuring concepts, while focus groups imply a quite different way of measuring concepts. Your design choices will play an important role in shaping how you measure your concepts.
Operationalizing your variables
Moving from identifying concepts to conceptualizing them and then to operationalizing them is a matter of increasing specificity. You begin the research process with a general interest, identify a few concepts that are essential for studying that interest you, work to define those concepts, and then spell out precisely how you will measure those concepts. In quantitative research, that final stage is called operationalization.
An operational definition consists of the following components: (1) the variable being measured, (2) the measure you will use, (3) how you plan to interpret the results of that measure.
The first component, the variable, should be the easiest part. In much quantitative research, there is a research question that has at least one independent and at least one dependent variable. Remember that variables have to be able to vary. For example, the United States is not a variable. Country of birth is a variable, as is patriotism. Similarly, if your sample only includes men, gender is a constant in your study…not a variable.
Let’s pick a social work research question and walk through the process of operationalizing variables. Suppose we hypothesize that individuals on a residential psychiatric unit who are more depressed are less likely to be satisfied with care than those who are less depressed. Remember, this would be a negative relationship—as depression increases, satisfaction decreases. In this question, depression is the independent variable (the cause) and satisfaction with care is the dependent variable (the effect). We have our two variables—depression and satisfaction with care—so the first component is done. Now, we move onto the second component–the measure.
How do you measure depression or satisfaction? Many students begin by thinking that they could look at body language to see if a person were depressed. Maybe they would also verbally express feelings of sadness or hopelessness more often. A satisfied person might be happy around service providers and express gratitude more often. These may indicate depression, but they lack coherence. Unfortunately, what this “measure” is actually saying is that “I know depression and satisfaction when I see them.” While you are likely a decent judge of depression and satisfaction, you need to provide more information in a research study for how you plan to measure your variables. Your judgment is subjective, based on your own idiosyncratic experiences with depression and satisfaction. They couldn’t be replicated by another researcher. They also can’t be done consistently for a large group of people. Operationalization requires that you come up with a specific and rigorous measure for seeing who is depressed or satisfied.
Finding a good measure for your variable can take less than a minute. To measure a variable like age, you would probably put a question on a survey that asked, “How old are you?” To evaluate someone’s length of stay in a hospital, you might ask for access to their medical records and count the days from when they were admitted to when they were discharged. Measuring a variable like income might require some more thought, though. Are you interested in this person’s individual income or the income of their family unit? This might matter if your participant does not work or is dependent on other family members for income. Do you count income from social welfare programs? Are you interested in their income per month or per year? Measures must be specific and clear.
Depending on your research design, your measure may be something you put on a survey or pre/post-test that you give to your participants. For a variable like age or income, one well-worded question may suffice. Unfortunately, most variables in the social world are not so simple. Depression and satisfaction are multi-dimensional variables, as they each contain multiple elements. Asking someone “Are you depressed?” does not do justice to the complexity of depression, which includes issues with mood, sleeping, eating, relationships, and happiness. Asking someone “Are you satisfied with the services you received?” similarly omits multiple dimensions of satisfaction, such as timeliness, respect, meeting needs, and likelihood of recommending to a friend, among many others.
INDICES, SCALES, AND TYPOLOGIES
To account for a variable’s dimensions, a researcher might rely on an index, scale, or typology. An index is a type of measure that contains several indicators and is used to summarize some more general concept. An index of depression might ask if the person has experienced any of the following indicators in the past month: pervasive feelings of hopelessness, thoughts of suicide, over- or under-eating, and a lack of enjoyment in normal activities. On their own, some of these indicators like over- or under-eating might not be considered depression, but collectively, the answers to each of these indicators add up to an overall experience of depression. The index allows the researcher in this case to better understand what shape a respondent’s depression experience takes. If the researcher had only asked whether a respondent had ever experienced depression, she wouldn’t know what sorts of behaviors actually made up that respondent’s experience of depression.
Taking things one step further, if the researcher decides to rank order the various behaviors that make up depression, perhaps weighting suicidal thoughts more heavily than eating disturbances, then she will have created a scale rather than an index. Like an index, a scale is also a measure composed of multiple items or questions. But unlike indexes, scales are designed in a way that accounts for the possibility that different items may vary in intensity.
If creating your own scale sounds complicated, don’t worry! For most variables, this work has already been done by other researchers. You do not need to create a scale for depression because scales such as the Patient Health Questionnaire (PHQ-9) and the Center for Epidemiologic Studies Depression Scale (CES-D) and Beck’s Depression Inventory (BDI) have been developed and refined over dozens of years to measure variables like depression. Similarly, scales such as the Patient Satisfaction Questionnaire (PSQ-18) have been developed to measure satisfaction with medical care. As we will discuss in the next section, these scales have been shown to be reliable and valid. While you could create a new scale to measure depression or satisfaction, a study with rigor would pilot test and refine that scale over time to make sure it measures the concept accurately and consistently. This high level of rigor is often unachievable in student research projects, so using existing scales is recommended.
Another reason existing scales are preferable is that they can save time and effort. The Mental Measurements Yearbook provides a searchable database of measures for different variables. You can access this database from your library’s list of databases. At the University of Texas at Arlington, the Mental Measurements Yearbook can be searched directly or viewed online. If you can’t find anything in there, your next stop should be the methods section of the articles in your literature review. The methods section of each article will detail how the researchers measured their variables. In a quantitative study, researchers likely used a scale to measure key variables and will provide a brief description of that scale. A Google Scholar search such as “depression scale” or “satisfaction scale” should also provide some relevant results. As a last resort, a general web search may bring you to a scale for your variable.
Unfortunately, all of these approaches do not guarantee that you will be able to actually see the scale itself or get information on how it is interpreted. Many scales cost money to use and may require training to properly administer. You may also find scales that are related to your variable but would need to be slightly modified to match your study’s needs. Adapting a scale to fit your study is a possibility; however, you should remember that changing even small parts of a scale can influence its accuracy and consistency. Pilot testing is always recommended for adapted scales.
A final way of measuring multidimensional variables is a typology. A typology is a way of categorizing concepts according to particular themes. Probably the most familiar version of a typology is the micro, meso, macro framework. Students classify specific elements of the social world by their ecological relationship with the person. Let’s take the example of depression again. The lack of sleep associated with depression would be classified as a micro-level element while a severe economic recession would be classified as a macro-level element. Typologies require clearly stated rules on what data will get assigned to what categories, so carefully following the rules of the typology is important.
Once you have (1) your variable and (2) your measure, you will need to (3) describe how you plan to interpret your measure. Sometimes, interpreting a measure is incredibly easy. If you ask someone their age, you’ll probably interpret the results by noting the raw number (e.g., 22) someone provides. However, you could also re-code age into categories (e.g., under 25, 20-29-years-old, etc.). An index may also be simple to interpret. If there is a checklist of problem behaviors, one might simply add up the number of behaviors checked off–with a higher total indicating worse behavior. Sometimes an index will assign people to categories (e.g., normal, borderline, moderate, significant, severe) based on their total number of checkmarks. As long as the rules are clearly spelled out, you are welcome to interpret measures in a way that makes sense to you. Theory might guide you to use some categories or you might be influenced by the types of statistical tests you plan to run later on in data analysis.
For more complicated measures like scales, you should look at the information provided by the scale’s authors for how to interpret the scale. If you can’t find enough information from the scale’s creator, look at how the results of that scale are reported in the results section of research articles. For example, Beck’s Depression Inventory (BDI-II) uses 21 questions to measure depression. A person indicates on a scale of 0-3 how much they agree with a statement. The results for each question are added up, and the respondent is put into one of three categories: low levels of depression (1-16), moderate levels of depression (17-30), or severe levels of depression (31 and over).
In sum, operationalization specifies what measure you will be using to measure your variable and how you plan to interpret that measure. Operationalization is probably the trickiest component of basic research methods. Don’t get frustrated if it takes a few drafts and a lot of feedback to get to a workable definition.
Qualitative research and operationalization
As we discussed in the previous section, qualitative research takes a more open approach towards defining the concepts in your research question. The questions you choose to ask in your interview, focus group, or content analysis will determine what data you end up getting from your participants. For example, if you are researching depression qualitatively, you would not use a scale like the Beck’s Depression Inventory, which is a quantitative measure we described above. Instead, you would start off with a tentative definition of what depression means based on your literature review and use that definition to come up with questions for your participants. We will cover how those questions fit into qualitative research designs later on in the textbook. For now, remember that qualitative researchers use the questions they ask participants to measure their variables and that qualitative researchers can change their questions as they gather more information from participants. Ultimately, the concepts in a qualitative study will be defined by the researcher’s interpretation of what her participants say. Unlike in quantitative research in which definitions must be explicitly spelled out in advance, qualitative research allows the definitions of concepts to emerge during data analysis.
Spotlight on UTA School of Social Work
Are interactions with a social robot associated with changes in depression and loneliness?
Robust measurement is very important in research. Furthermore, providing a clear explanation of the measures used in a study helps others to understand the concepts being studied and interpret the findings as well as and helps other researchers to accurately replicate the study in different settings.
Dr. Noelle Fields and Ling Xu from the University of Texas at Arlington’s School of Social Work collaborated with Dr. Julienne Greer from the College of Liberal Arts on a pilot study that incorporated a participatory arts intervention with the social robot, NAO. The intervention took place with older adults living in an assisted living facility. The overall aim of this study was to help older adults improve their psychological well-being through participation in a theatre arts activity led by NAO.
The key outcome variables for this pilot study were psychological well-being measured by depression, loneliness, and engagement with the robot. Depression and loneliness were measured by two standardized scales: the 15-item Geriatric Depression Scale (Sheikh & Yesavage, 1986) and the revised 3-item UCLA loneliness scale (Hughes, Waite, Hawkley, & Cacioppo, 2004). However, engagement with the robot did not have a standardized measure. Thus, the research team utilized a measure to capture engagement with the robot based on previous research.
In this study, engagement with robot was defined as the degree of interaction or involvement with a robot. One way to measure engagement is for members of the research team (i.e., observers) to rate the level of participant engagement (see Table 1).
Table 1. Please circle 0-5 to indicate the participant’s engagement levels (definitions for each levels can be found in the example column).
Rating | Meaning | Example |
0 | Intense noncompliance | Participant stood and walked away from the table on which the robot interaction took place |
1 | Noncompliance | Participant hung head and refused to comply with interviewer’s request to speak to the robot |
2 | Neutral | Participant complied with instructions to speak with the robot after several prompts from the confederate |
3 | Slight interest | Participant required two or three prompts from the confederate before responding to the robot |
4 | Engagement | Participant complied immediately following the confederate’s request to speak with the robot |
5 | Intense engagement | Participant spontaneously engaged with the robot |
This measurement was easy to apply in this study; however, it may lack the sensitivity to capture more detailed information about engagement, especially among older adult populations. Therefore, the researchers in this pilot study designed additional indicators to describe the participants’ reactions when interacting with a robot. More specifically, after watching a video of each participant interacting with NAO, each researcher gave an engagement score based on the following concepts: (1) attentiveness including focus on face of robot or gesture of robot, (2) smiling and/or laughter, (3) head nodding, and (4) facial/vocal expression that included eyes widening, eyebrows arching, and tonal changes in voice. Through video analysis, each of the concepts were counted and tabulated by independent researchers, and mean score among researchers on each concept was then calculated. Sum scores on total engagement were also adapted for analysis. See Table 2 for detailed information of this measurement.
Table 2: Each researcher should provide a score on each item below based on your observation of participants’ interaction with the robot.
1.Strongly disagree | 2. Disagree | 3. Neither agree or disagree | 4. Agree | 5.Strongly agree | |
Attentive | |||||
a. focus on face of robot | |||||
b. focus on gesture of robot | |||||
Smiling and/or laughter | |||||
Head nodding | |||||
Facial/Vocal expression | |||||
a. Eyes widen | |||||
b. Eyebrow arch | |||||
c. Tonal changes in voice |
The study found that participants reported improvements in mood, loneliness, and depression. The degree of difference/change was slightly greater in participants without dementia, perhaps suggesting social engagement and connection was a more profound attribute in cognitively intact older adults. Further research would be needed to confirm this hypothesis. Although the study is limited by its small scale and non-intervention control group, this exploratory pilot study supports the continuing development of participatory arts interventions with older adults using a social robotic platform. The benefits of performative participatory art between social robots and older adults is an emerging research area for human-robot social interactions and communications.
Key Takeaways
- Operationalization involves spelling out precisely how a concept will be measured.
- Operational definitions must include the variable, the measure, and how you plan to interpret the measure.
- Multi-dimensional concepts can be measured by an index, a scale, or a typology.
- It’s a good idea to look at how researchers have measured the concept in previous studies.
Glossary
- Index- measure that contains several indicators and is used to summarize a more general concept
- Indicators- represent the concepts that we are interested in studying
- Operationalization- process by which researchers conducting quantitative research spell out precisely how a concept will be measured and how to interpret that measure
- Scale- composite measure designed in a way that accounts for the possibility that different items on an index may vary in intensity
- Typology- measure that categorizes concepts according to particular themes
5.5 Measurement quality
Learning Objectives
- Define reliability and describe the types of reliability
- Define validity and describe the types of validity
In quantitative research, once we’ve defined our terms and specified the operations for measuring them, how do we know that our measures are any good? Without some assurance of the quality of our measures, we cannot be certain that our findings have any meaning or, at the least, that our findings mean what we think they mean. When social scientists measure concepts, they aim to achieve reliability and validity in their measures. These two aspects of measurement quality are the focus of this section. We’ll consider reliability first and then take a look at validity. For both aspects of measurement quality, let’s say our interest is in measuring the concepts of alcoholism and alcohol intake. What are some potential problems that could arise when attempting to measure this concept, and how might we work to overcome those problems?
Reliability
First, let’s say we’ve decided to measure alcoholism by asking people to respond to the following question: Have you ever had a problem with alcohol? If we measure alcoholism in this way, it seems likely that anyone who identifies as an alcoholic would respond with a yes to the question. So, this must be a good way to identify our group of interest, right? Well, maybe. Think about how you or others you know would respond to this question. Would responses differ after a wild night out from what they would have been the day before? Might an infrequent drinker’s current headache from the single glass of wine she had last night influence how she answers the question this morning? How would that same person respond to the question before consuming the wine? In each of these cases, if the same person would respond differently to the same question at different points, it is possible that our measure of alcoholism has a reliability problem. Reliability in measurement is about consistency.
One common problem of reliability with social scientific measures is memory. If we ask research participants to recall some aspect of their own past behavior, we should try to make the recollection process as simple and straightforward for them as possible. Sticking with the topic of alcohol intake, if we ask respondents how much wine, beer, and liquor they’ve consumed each day over the course of the past 3 months, how likely are we to get accurate responses? Unless a person keeps a journal documenting their intake, there will very likely be some inaccuracies in their responses. If, on the other hand, we ask a person how many drinks of any kind they have consumed in the past week, we might get a more accurate set of responses.
Reliability can be an issue even when we’re not reliant on others to accurately report their behaviors. Perhaps a researcher is interested in observing how alcohol intake influences interactions in public locations. She may decide to conduct observations at a local pub, noting how many drinks patrons consume and how their behavior changes as their intake changes. But what if the researcher has to use the restroom and misses the three shots of tequila that the person next to her downs during the brief period she is away? The reliability of this researcher’s measure of alcohol intake, counting numbers of drinks she observes patrons consume, depends on her ability to actually observe every instance of patrons consuming drinks. If she is unlikely to be able to observe every such instance, then perhaps her mechanism for measuring this concept is not reliable.
If a measure is reliable, it means that if the measure is given multiple times, the results will be consistent each time. For example, if you took the SATs on multiple occasions before coming to school, your scores should be relatively the same from test to test. This is what is known as test-retest reliability. In the same way, if a person is clinically depressed, a depression scale should give similar (though not necessarily identical) results today that it does two days from now.
If your study involves observing people’s behaviors, for example watching sessions of mothers playing with infants, you may also need to assess inter-rater reliability. Inter-rater reliability is the degree to which different observers agree on what happened. Did you miss when the infant offered an object to the mother and the mother dismissed it? Did the other person rating miss that event? Do you both similarly rate the parent’s engagement with the child? Again, scores of multiple observers should be consistent, though perhaps not perfectly identical.
Finally, for scales, internal consistency reliability is an important concept. The scores on each question of a scale should be correlated with each other, as they all measure parts of the same concept. Think about a scale of depression, like Beck’s Depression Inventory. A person who is depressed would score highly on most of the measures, but there would be some variation. If we gave a group of people that scale, we would imagine there should be a correlation between scores on, for example, mood disturbance and lack of enjoyment. They aren’t the same concept, but they are related. So, there should be a mathematical relationship between them. A specific statistic known as Cronbach’s Alpha provides a way to measure how well each question of a scale is related to the others. Cronbach’s alpha (sometimes shown as α) can range from 0 to 1.0. As a general rule, Cronbach’s alpha should be at least .7 to reflect acceptable internal consistency with scores of .8 or higher considered an indicator of good internal consistency.
Test-retest, inter-rater, and internal consistency are three important subtypes of reliability. Researchers use these types of reliability to make sure their measures are consistently measuring the concepts in their research questions.
Validity
While reliability is about consistency, validity is about accuracy. What image comes to mind for you when you hear the word alcoholic? Are you certain that the image you conjure up is similar to the image others have in mind? If not, then we may be facing a problem of validity.
For a measure to have validity, we must be certain that our measures accurately get at the meaning of our concepts. Think back to the first possible measure of alcoholism we considered in the previous few paragraphs. There, we initially considered measuring alcoholism by asking research participants the following question: Have you ever had a problem with alcohol? We realized that this might not be the most reliable way of measuring alcoholism because the same person’s response might vary dramatically depending on how they are feeling that day. Likewise, this measure of alcoholism is not particularly valid. What is “a problem” with alcohol? For some, it might be having had a single regrettable or embarrassing moment that resulted from consuming too much. For others, the threshold for “problem” might be different; perhaps a person has had numerous embarrassing drunken moments but still gets out of bed for work every day, so they don’t perceive themselves as having a problem. Because what each respondent considers to be problematic could vary so dramatically, our measure of alcoholism isn’t likely to yield any useful or meaningful results if our aim is to objectively understand, say, how many of our research participants have alcoholism. [1]
Types of validity
Below are some basic subtypes of validity, though there are certainly others you can read more about. One way to think of validity is to think of it as you would a portrait. Some portraits of people look just like the actual person they are intended to represent. But other representations of people’s images, such as caricatures and stick drawings, are not nearly as accurate. While a portrait may not be an exact representation of how a person looks, what’s important is the extent to which it approximates the look of the person it is intended to represent. The same goes for validity in measures. No measure is exact, but some measures are more accurate than others.
Face validity
In the last paragraph, critical engagement with our measure for alcoholism “Do you have a problem with alcohol?” was shown to be flawed. We assessed its face validity or whether it is plausible that the question measures what it intends to measure. Face validity is a subjective process. Sometimes face validity is easy, as a question about height wouldn’t have anything to do with alcoholism. Other times, face validity can be more difficult to assess. Let’s consider another example.
Perhaps we’re interested in learning about a person’s dedication to healthy living. Most of us would probably agree that engaging in regular exercise is a sign of healthy living, so we could measure healthy living by counting the number of times per week that a person visits their local gym. But perhaps they visit the gym to use their tanning beds or to flirt with potential dates or sit in the sauna. These activities, while potentially relaxing, are probably not the best indicators of healthy living. Therefore, recording the number of times a person visits the gym may not be the most valid way to measure their dedication to healthy living.
Content validity
Another problem with this measure of healthy living is that it is incomplete. Content validity assesses for whether the measure includes all of the possible meanings of the concept. Think back to the previous section on multidimensional variables. Healthy living seems like a multidimensional concept that might need an index, scale, or typology to measure it completely. Our one question on gym attendance doesn’t cover all aspects of healthy living. Once you have created one, or found one in the existing literature, you need to assess for content validity. Are there other aspects of healthy living that aren’t included in your measure?
Criterion validity
Let’s say you have created (or found) a good scale, index, or typology for your measure of healthy living. A valid measure of healthy living would have scores that are similar to other measures of healthy living. Criterion validity occurs when the results from the measure are similar to those from an external criterion (that, ideally, has already been validated or is a more direct measure of the variable).
There are two types of criterion validity — predictive validity and concurrent validity. They are distinguished by timing — whether or not the measure is similar to something that is measured in the future or at the same time. Predictive validity means that your measure predicts things it should be able to predict. A valid measure of healthy living would be able to predict, for example, scores of a blood panel test during a patient’s annual physical. In this case, the assumption is that if you have a healthy lifestyle, a standard blood test done a few months later during an annual checkup would show healthy results. On the other hand, if we were to administer the blood panel measure at the same time as the scale of healthy living, we would be assessing concurrent validity. Concurrent validity is the same as predictive validity—the scores on your measure should be similar to an established measure—except that both measures are given at the same time.
Construct validity
Another closely related concept is construct validity. The logic behind construct validity is that sometimes there is no established criterion to use for comparison. However, there may be a construct that is theoretically related to the variable being measured. The measure could then be compared to that construct, even though it isn’t exactly the same concept as what’s being measured. In other words, construct validity exists when the measure is related to other measures that are hypothesized to be related to it.
It might be helpful to look at two types of construct validity – convergent validity and discriminant validity. Convergent validity takes an existing measure of the same concept and compares your measure to it. If their scores are similar, then it’s probably likely that they are both measuring the same concept.In assessing for convergent validity, one should look for different methods of measuring the same concept. If someone filled out a scale about their substance use and the results from the self-reported scale consistently matched the results of a lab test, then the scale about substance use would demonstrate convergent validity. Discriminant validity is a similar concept, except you would be comparing your measure to one that is expected to be unrelated. A participant’s scores on a healthy lifestyle measure shouldn’t be too closely correlated with a scale that measures self-esteem because you want the measure to discriminate between the two constructs.
Reliability versus Validity
If you are still confused about validity and reliability, Figure 5.2 shows an example of what validity and reliability look like. On the first target, our shooter’s aim is all over the place. It is neither reliable (consistent) nor valid (accurate). The second (middle) target demonstrates consistency…but it is reliably off-target, or invalid. The third and final target (bottom right) represents a reliable and valid result. The person is able to hit the target accurately and consistently. This is what you should aim for in your research. An instrument can be reliable without being valid (target 2), but it cannot be valid without also being reliable (target 3).
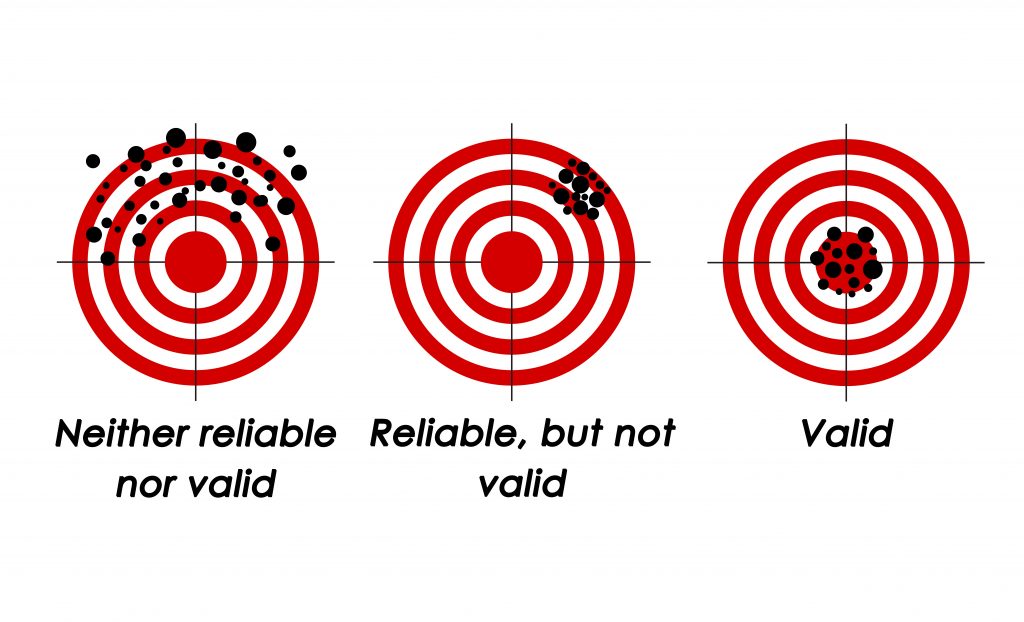
Key Takeaways
- Reliability is a matter of consistency.
- Validity is a matter of accuracy.
- There are many types of validity and reliability.
Glossary
- Concurrent validity- if a measure is able to predict outcomes from an established measure given at the same time
- Content validity- if the measure includes all of the possible meanings of the concept
- Convergent validity- if a measure is conceptually similar to an existing measure of the same concept
- Discriminant validity- if a measure is not related to measures to which it shouldn’t be statistically correlated
- Face validity- if it is plausible that the measure measures what it intends to
- Internal consistency reliability- degree to which scores on each question of a scale are correlated with each other
- Inter-rater reliability- the degree to which different observers agree on what happened
- Predictive validity- if a measure predicts things it should be able to predict in the future
- Reliability- a measure’s consistency.
- Test-retest reliability- if a measure is given multiple times, the results will be consistent each time
- Validity- a measure’s accuracy
5.6 Challenges in quantitative measurement
Learning Objectives
- Identify potential sources of error
- Differentiate between systematic and random error
For quantitative methods, you should now have some idea about how conceptualization and operationalization work, and you should also know how to assess the quality of your measures. But measurement is sometimes a complex process, and some concepts are more complex than others. Measuring a person’s political party affiliation, for example, is less complex than measuring their sense of alienation. In this section, we’ll consider some of these complexities in measurement.
Systematic error
Unfortunately, measures never perfectly describe what exists in the real world. Good measures demonstrate reliability and validity but will always have some degree of error. Systematic error causes our measures to consistently output incorrect data, usually due to an identifiable process. Imagine you created a measure of height, but you didn’t put an option for anyone over six feet tall. If you gave that measure to your local college or university, some of the taller members of the basketball team might not be measured accurately. In fact, you would be under the mistaken impression that the tallest person at your school was six feet tall, when in actuality there are likely plenty of people taller than six feet at your school. This error seems innocent, but if you were using that measure to help you build a new building, those people might hit their heads!
A less innocent form of error arises when researchers using question wording that might cause participants to think one answer choice is preferable to another. For example, someone were to ask you, “Do you think global warming is caused by human activity?” you would probably feel comfortable answering honestly. But what if someone asked you, “Do you agree with 99% of scientists that global warming is caused by human activity?” Would you feel comfortable saying no, if that’s what you honestly felt? Possibly not. That is an example of a leading question, a question with wording that influences how a participant responds. We’ll discuss leading questions and other problems in question wording in greater detail in Chapter 7.
In addition to error created by the researcher, participants can cause error in measurement. Some people will respond without fully understanding a question, particularly if the question is worded in a confusing way. That’s one source of error. Let’s consider another. If we asked people if they always washed their hands after using the bathroom, would we expect people to be perfectly honest? Polling people about whether they wash their hands after using the bathroom might only elicit what people would like others to think they do, rather than what they actually do. This is an example of social desirability bias, in which participants in a research study want to present themselves in a positive, socially desirable way to the researcher. People in your study will want to seem tolerant, open-minded, and intelligent, but their true feelings may be closed-minded, simple, and biased. So, they lie. This occurs often in political polling, which may show greater support for a candidate from a minority race, gender, or political party than actually exists in the electorate.
A related form of bias is called acquiescence bias, also known as “yea-saying.” It occurs when people say yes to whatever the researcher asks, even when doing so contradicts previous answers. For example, a person might say yes to both “I am a confident leader in group discussions” and “I feel anxious interacting in group discussions.” Those two responses are unlikely to both be true for the same person. Why would someone do this? Similar to social desirability, people want to be agreeable and nice to the researcher asking them questions or they might ignore contradictory feelings when responding to each question. Respondents may also act on cultural reasons, trying to “save face” for themselves or the person asking the questions. Regardless of the reason, the results of your measure don’t match what the person truly feels.
Random error
So far, we have discussed sources of error that come from choices made by respondents or researchers. Usually, systematic errors will result in responses that are incorrect in one direction or another. For example, social desirability bias usually means more people will say they will vote for a third party in an election than actually do. Systematic errors such as these can be reduced, but there is another source of error in measurement that can never be eliminated, and that is random error. Unlike systematic error, which biases responses consistently in one direction or another, random error is unpredictable and does not consistently result in scores that are consistently higher or lower on a given measure. Instead, random error is more like statistical noise, which will likely average out across participants.
Random error is present in any measurement. If you’ve ever stepped on a bathroom scale twice and gotten two slightly different results, then you’ve experienced random error. Maybe you were standing slightly differently or had a fraction of your foot off of the scale the first time. If you were to take enough measures of your weight on the same scale, you’d be able to figure out your true weight. In social science, if you gave someone a scale measuring depression on a day after they lost their job, they would likely score differently than if they had just gotten a promotion and a raise. Even if the person were clinically depressed, our measure is subject to influence by the random occurrences of life. Thus, social scientists speak with humility about our measures. We are reasonably confident that what we found is true, but we must always acknowledge that our measures are only an approximation of reality.
Humility is important in scientific measurement, as errors can have real consequences. When Matthew DeCarlo was writing the source material for this book, he and his wife were expecting their first child. Like most people, they used a pregnancy test from the pharmacy. If the test said his wife was pregnant when she was not, that would be a false positive. On the other hand, if the test indicated that she was not pregnant when she was in fact pregnant, that would be a false negative. Even if the test is 99% accurate, that means that one in a hundred women will get an erroneous result when they use a home pregnancy test. For them, a false positive would have been initially exciting, then devastating when they found out they were not having a child. A false negative would have been disappointing at first and then quite shocking when they found out they were indeed having a child. While both false positives and false negatives are not very likely for home pregnancy tests (when taken correctly), measurement error can have consequences for the people being measured.
Key Takeaways
- Systematic error may arise from the researcher, participant, or measurement instrument.
- Systematic error biases results in a particular direction, whereas random error can be in any direction.
- All measures are prone to error and should interpreted with humility.
Glossary
- Acquiescence bias- when respondents say yes to whatever the researcher asks
- False negative- when a measure does not indicate the presence of a phenomenon, when in reality it is present
- False positive- when a measure indicates the presence of a phenomenon, when in reality it is not present
- Leading question- a question with wording that influences how a participant responds
- Random error- unpredictable error that does not consistently result in scores that are consistently higher or lower on a given measure
- Social desirability bias- when respondents answer based on what they think other people would like, rather than what is true
- Systematic error- measures consistently output incorrect data, usually in one direction and due to an identifiable process