Chapter 2 oriented you to the theories relevant to your topic area; the macro, meso, or micro levels of analysis; and the assumptions or paradigms of research. This chapter will use these elements to help you conceptualize and design your research project. You will make specific choices about the purpose of your research, quantitative or qualitative methods, and establishing causality. You’ll also learn how and why researchers use both qualitative and quantitative methods in the same study.
Chapter Outline
- 4.1 Types of research
- 4.2 Causality
- 4.3 Unit of analysis and unit of observation
- 4.4 Mixed methods
Content Advisory
This chapter discusses or mentions the following topics: child neglect and abuse, sexual harassment, the criminal justice system, homelessness, sexual and domestic violence, depression, and substance abuse.
4.1 Types of research
Learning Objectives
- Differentiate between exploratory, descriptive, and explanatory research
A recent news story about college students’ addictions to electronic gadgets (Lisk, 2011) describes findings from some research by Professor Susan Moeller and colleagues from the University of Maryland. The story raises a number of interesting questions. Just what sorts of gadgets are students addicted to? How do these addictions work? Why do they exist, and who is most likely to experience them?
Social science research is great for answering just these sorts of questions. But in order to answer our questions well, we must take care in designing our research projects. In this chapter, we’ll consider what aspects of a research project should be considered at the beginning, including specifying the goals of the research, the components that are common across most research projects, and a few other considerations.
One of the first things to think about when designing a research project is what you hope to accomplish, in very general terms, by conducting the research. What do you hope to be able to say about your topic? Do you hope to gain a deep understanding of whatever phenomenon it is that you’re studying, or would you rather have a broad, but perhaps less deep, understanding? Do you want your research to be used by policymakers or others to shape social life, or is this project more about exploring your curiosities? Your answers to each of these questions will shape your research design.
Exploration, description, and explanation
You’ll need to decide in the beginning phases whether your research will be exploratory, descriptive, or explanatory. Each has a different purpose, so how you design your research project will be determined in part by this decision.
Researchers conducting exploratory research are typically at the early stages of examining their topics. These sorts of projects are usually conducted when a researcher wants to test the feasibility of conducting a more extensive study and to figure out the “lay of the land” with respect to the particular topic. Perhaps very little prior research has been conducted on this subject. If this is the case, a researcher may wish to do some exploratory work to learn what method to use in collecting data, how best to approach research subjects, or even what sorts of questions are reasonable to ask. A researcher wanting to simply satisfy her own curiosity about a topic could also conduct exploratory research. In the case of the study of college students’ addictions to their electronic gadgets, a researcher conducting exploratory research on this topic may simply wish to learn more about students’ use of these gadgets. Because these addictions seemed to be a relatively new phenomenon, an exploratory study of the topic made sense as an initial first step toward understanding it.
It is important to note that exploratory designs do not make sense for topic areas with a lot of existing research. For example, the question “What are common interventions for parents who neglect their children?” would not make much sense as a research question. One could simply look at journal articles and textbooks to see what interventions are commonly used with this population. Exploratory questions are best suited to topics that have not been studied. Students may sometimes say there is not much literature on their chosen topic, when there is in fact a large body of literature on that topic. However, that said, there are a few students each semester who pick a topic for which there is little existing research. Perhaps, if you were looking at child neglect interventions for parents who identify as transgender or parents who are refugees from the Syrian civil war, less would be known about child neglect for those specific populations. In that case, an exploratory design would make sense as there is less literature to guide your study.
Descriptive research is used to describe or define a particular phenomenon. For example, a social work researcher may want to understand what it means to be a first-generation college student or a resident in a psychiatric group home. In this case, descriptive research would be an appropriate strategy. A descriptive study of college students’ addictions to their electronic gadgets, for example, might aim to describe patterns in how many hours students use gadgets or which sorts of gadgets students tend to use most regularly.
Researchers at the Princeton Review conduct descriptive research each year when they set out to provide students and their parents with information about colleges and universities around the United States. They describe the social life at a school, the cost of admission, and student-to-faculty ratios (to name just a few of the categories reported). Although students and parents may be able to obtain much of this information on their own, having access to the data gathered by a team of researchers is much more convenient and less time consuming.
Social workers often rely on descriptive research to tell them about their service area. Keeping track of the number of children receiving foster care services, their demographic makeup (e.g., race, gender), and length of time in care are excellent examples of descriptive research. On a more macro-level, the Centers for Disease Control provides a remarkable amount of descriptive research on mental and physical health conditions. In fact, descriptive research has many useful applications, and you probably rely on findings from descriptive research without even being aware that that is what you are doing.
Finally, social work researchers often aim to explain why particular phenomena work in the way that they do. Research that answers “why” questions is referred to as explanatory research. In this case, the researcher is trying to identify the causes and effects of whatever phenomenon she is studying. An explanatory study of college students’ addictions to their electronic gadgets might aim to understand why students become addicted. Does it have anything to do with their family histories? With their other extracurricular hobbies and activities? With whom they spend their time? An explanatory study could answer these kinds of questions.
There are numerous examples of explanatory social scientific investigations. For example, in one study, Dominique Simons and Sandy Wurtele (2010) sought to discover whether receiving corporal punishment from parents led children to turn to violence in solving their interpersonal conflicts with other children. In their study of 102 families with children between the ages of 3 and 7, the researchers found that experiencing frequent spanking did, in fact, result in children being more likely to accept aggressive problem-solving techniques. Another example of explanatory research can be seen in Robert Faris and Diane Felmlee’s (2011) research on the connections between popularity and bullying. From their study of 8th, 9th, and 10th graders in 19 North Carolina schools, they found that aggression increased as adolescents’ popularity increased. (This pattern was found until adolescents reached the top 2% in the popularity ranks. After that, aggression declines).
The choice between descriptive, exploratory, and explanatory research should be made with your research question in mind. What does your question ask? Are you trying to learn the basics about a new area, establish a clear “why” relationship, or define or describe an activity or concept? In the next section, we will explore how each type of research is associated with different methods, paradigms, and forms of logic.
Key Takeaways
- Exploratory research is usually conducted when a researcher has just begun an investigation and wishes to understand the topic generally.
- Descriptive research is research that aims to describe or define the topic at hand.
- Explanatory research is research that aims to explain why particular phenomena work in the way that they do.
Glossary
- Descriptive research- research that describes or define a particular phenomenon
- Explanatory research- explains why particular phenomena work in the way that they do, answers “why” questions
- Exploratory research- conducted during the early stages of a project, usually when a researcher wants to test the feasibility of conducting a more extensive study
4.2 Causality
Learning Objectives
- Define and provide an example of idiographic and nomothetic causal explanations
- Describe the role of causality in quantitative research as compared to qualitative research
- Identify, define, and describe each of the main criteria for nomothetic causal explanations
- Describe the difference between and provide examples of independent, dependent, and control variables
- Define hypothesis, be able to state a clear hypothesis, and discuss the respective roles of quantitative and qualitative research when it comes to hypotheses
Most social scientific studies attempt to provide some kind of causal explanation. In other words, it is about cause and effect. A study on an intervention to prevent child abuse is trying to draw a connection between the intervention and changes in child abuse. Causality refers to the idea that one event, behavior, or belief will result in the occurrence of another, subsequent event, behavior, or belief. It seems simple, but you may be surprised to learn there is more than one way to explain how one thing causes another. How can that be? How could there be many ways to understand causality?
Think back to our chapter on paradigms, which were analytic lenses comprised of assumptions about the world. You’ll remember the positivist paradigm as the one that believes in objectivity and social constructionist paradigm as the one that believes in subjectivity. Both paradigms are correct, though incomplete, viewpoints on the social world and social science.
A researcher operating in the social constructionist paradigm would view truth as subjective. In causality, that means that in order to try to understand what caused what, we would need to report what people tell us. Well, that seems pretty straightforward, right? Well, what if two different people saw the same event from the exact same viewpoint and came up with two totally different explanations about what caused what? A social constructionist might say that both people are correct. There is not one singular truth that is true for everyone, but many truths created and shared by people.
When social constructionists engage in science, they are trying to establish one type of causality—idiographic causality. The word idiographic comes from the root word “idio” which means peculiar to one, personal, and distinct. An idiographiccausal explanation means that you will attempt to explain or describe your phenomenon exhaustively, based on the subjective understandings of your participants. Idiographic causal explanations are intended to explain one particular context or phenomenon. These explanations are bound with the narratives people create about their lives and experience, and are embedded in a cultural, historical, and environmental context. Idiographic causal explanations are so powerful because they convey a deep understanding of a phenomenon and its context. From a social constructionist perspective, the truth is messy. Idiographic research involves finding patterns and themes in the causal themes established by your research participants.
If that doesn’t sound like what you normally think of as “science,” you’re not alone. Although the ideas behind idiographic research are quite old in philosophy, they were only applied to the sciences at the start of the last century. If we think of famous Western scientists like Newton or Darwin, they never saw truth as subjective. They operated with the understanding there were objectively true laws of science that were applicable in all situations. In their time, another paradigm–the positivist paradigm–was dominant and continues its dominance today. When positivists try to establish causality, they are like Newton and Darwin, trying to come up with a broad, sweeping explanation that is universally true for all people. This is the hallmark of a nomothetic causal explanation. The word nomothetic is derived from the root word “nomo” which means related to a law or legislative, and “thetic” which means something that establishes. Put the root words together and it means something that is establishing a law, or in our case, a universal explanation.
Nomothetic causal explanations are incredibly powerful. They allow scientists to make predictions about what will happen in the future, with a certain margin of error. Moreover, they allow scientists to generalize—that is, make claims about a large population based on a smaller sample of people or items. Generalizing is important. We clearly do not have time to ask everyone their opinion on a topic, nor do we have the ability to look at every interaction in the social world. We need a type of causal explanation that helps us predict and estimate truth in all situations.
If these still seem like obscure philosophy terms, let’s consider an example. Imagine you are working for a community-based non-profit agency serving people with disabilities. You are putting together a report to help lobby the state government for additional funding for community support programs, and you need to support your argument for additional funding at your agency. If you looked at nomothetic research, you might learn how previous studies have shown that, in general, community-based programs like yours are linked with better health and employment outcomes for people with disabilities. Nomothetic research seeks to explain that community-based programs are better for everyone with disabilities. If you looked at idiographic research, you would get stories and experiences of people in community-based programs. These individual stories are full of detail about the lived experience of being in a community-based program. Using idiographic research, you can understand what it’s like to be a person with a disability and then communicate that to the state government. For example, a person might say “I feel at home when I’m at this agency because they treat me like a family member” or “this is the agency that helped me get my first paycheck.”
Neither kind of causal explanation is better than the other. A decision to conduct idiographic research means that you will attempt to explain or describe your phenomenon exhaustively, attending to cultural context and subjective interpretations. A decision to conduct nomothetic research, on the other hand, means that you will try to explain what is true for everyone and predict what will be true in the future. In short, idiographic explanations have greater depth, and nomothetic explanations have greater breadth. More importantly, social workers understand the value of both approaches to understanding the social world. A social worker helping a client with substance abuse issues seeks idiographic knowledge when they ask about that client’s life story, investigate their unique physical environment, or probe how they understand their addiction. At the same time, a social worker also uses nomothetic knowledge to guide their interventions. Nomothetic research may help guide them to minimize risk factors and maximize protective factors or use an evidence-based therapy, relying on knowledge about what in general helps people with substance abuse issues.
Nomothetic causal explanations
If you are trying to generalize about causality, or create a nomothetic causal explanation, then the rest of these statements are likely to be true: you will use quantitative methods, reason deductively, and engage in explanatory research. How can we make that prediction? Let’s take it part by part.
Because nomothetic causal explanations try to generalize, they must be able to reduce phenomena to a universal language, mathematics. Mathematics allows us to precisely measure, in universal terms, phenomena in the social world. Because explanatory researchers want a clean “x causes y” explanation, they need to use the universal language of mathematics to achieve their goal. That’s why nomothetic causal explanations use quantitative methods. It’s helpful to note that not all quantitative studies are explanatory. For example, a descriptive study could reveal the number of people without homes in your county, though it won’t tell you why they are homeless. But nearly all explanatory studies are quantitative.
What we’ve been talking about here is an association between variables. When one variable precedes or predicts another, we have what researchers call independent and dependent variables. Two variables can be associated without having a causal relationship. However, when certain conditions are met (which we describe later in this chapter), the independent variable is considered as a “cause” of the dependent variable. For our example on spanking and aggressive behavior, spanking would be the independent variable and aggressive behavior addiction would be the dependent variable. In causal explanations, the independent variable is the cause, and the dependent variable is the effect. Dependent variables depend on independent variables. If all of that gets confusing, just remember this graphical depiction:
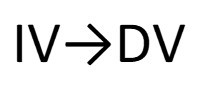
The strength of the association between the independent variable and dependent variable is another important factor to take into consideration when attempting to make causal claims when your research approach is nomothetic. In this context, strength refers to statistical significance. When the association between two variables is shown to be statistically significant, we can have greater confidence that the data from our sample reflect a true association between those variables in the target population. Statistical significance is usually represented in statistics as the p-value. Generally a p-value of .05 or less indicates the association between the two variables is statistically significant.
A hypothesis is a statement describing a researcher’s expectation regarding the research findings. Hypotheses in quantitative research are nomothetic causal explanations that the researcher expects to demonstrate. Hypotheses are written to describe the expected association between the independent and dependent variables. Your prediction should be taken from a theory or model of the social world. For example, you may hypothesize that treating clinical clients with warmth and positive regard is likely to help them achieve their therapeutic goals. That hypothesis would be using the humanistic theories of Carl Rogers. Using previous theories to generate hypotheses is an example of deductive research. If Rogers’ theory of unconditional positive regard is accurate, your hypothesis should be true.
Let’s consider a couple of examples. In research on sexual harassment (Uggen & Blackstone, 2004), one might hypothesize, based on feminist theories of sexual harassment, that more females than males will experience specific sexually harassing behaviors. What is the causal explanation being predicted here? Which is the independent and which is the dependent variable? In this case, we hypothesized that a person’s gender (independent variable) would predict their likelihood to experience sexual harassment (dependent variable).
Sometimes researchers will hypothesize that an association will take a specific direction. As a result, an increase or decrease in one area might be said to cause an increase or decrease in another. For example, you might choose to study the association between age and support for legalization of marijuana. Perhaps you’ve taken a sociology class and, based on the theories you’ve read, you hypothesize that age is negatively related to support for marijuana legalization. In fact, there are empirical data that support this hypothesis. Gallup has conducted research on this very question since the 1960s (Carroll, 2005). What have you just hypothesized? You have hypothesized that as people get older, the likelihood of their supporting marijuana legalization decreases. Thus, as age (your independent variable) moves in one direction (up), support for marijuana legalization (your dependent variable) moves in another direction (down). So, positive associations involve two variables going in the same direction and negative associations involve two variables going in opposite directions. If writing hypotheses feels tricky, it is sometimes helpful to draw them out and depict each of the two hypotheses we have just discussed.
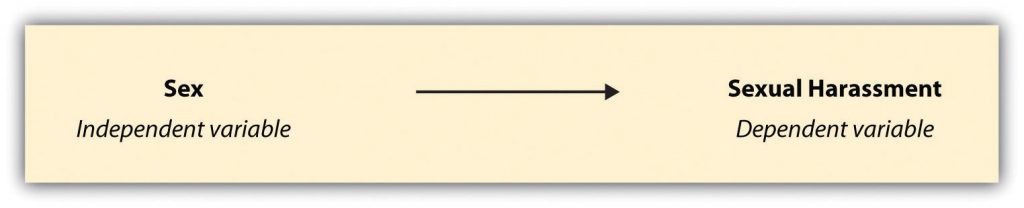
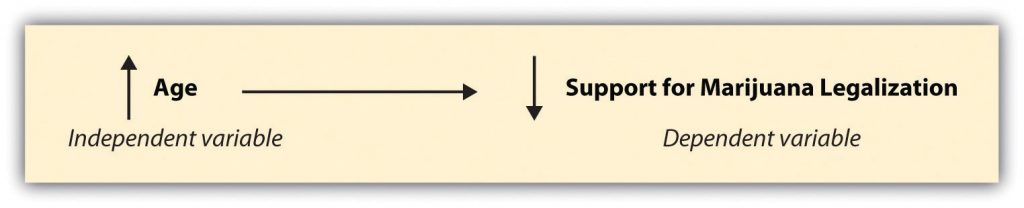
It’s important to note that once a study starts, it is unethical to change your hypothesis to match the data that you found. For example, what happens if you conduct a study to test the hypothesis from Figure 4.3 on support for marijuana legalization, but you find no association between age and support for legalization? It means that your hypothesis was wrong, but that’s still valuable information. It would challenge what the existing literature says on your topic, demonstrating that more research needs to be done to figure out the factors that impact support for marijuana legalization. Don’t be embarrassed by negative results, and definitely don’t change your hypothesis to make it appear correct all along!
Establishing causality in nomothetic research
Let’s say you conduct your study and you find evidence that supports your hypothesis, as age increases, support for marijuana legalization decreases. Success! Causal explanation complete, right? Not quite. You’ve only established one of the criteria for causality. The main criteria for causality have to do with covariation, plausibility, temporality, and spuriousness. In our example from Figure 4.3, we have established only one criteria—covariation. When variables covary, they vary together. Both age and support for marijuana legalization vary in our study. Our sample contains people of varying ages and varying levels of support for marijuana legalization and they vary together in a patterned way–when age increases, support for legalization decreases.
Just because there might be some correlation between two variables does not mean that a causal explanation between the two is really plausible. Plausibility means that in order to make the claim that one event, behavior, or belief causes another, the claim has to make sense. It makes sense that people from previous generations would have different attitudes towards marijuana than younger generations. People who grew up in the time of Reefer Madness or the hippies may hold different views than those raised in an era of legalized medicinal and recreational use of marijuana.
Once we’ve established that there is a plausible association between the two variables, we also need to establish that the cause happened before the effect, the criterion of temporality. A person’s age is a quality that appears long before any opinions on drug policy, so temporally the cause comes before the effect. It wouldn’t make any sense to say that support for marijuana legalization makes a person’s age increase. Even if you could predict someone’s age based on their support for marijuana legalization, you couldn’t say someone’s age was caused by their support for legalization.
Finally, scientists must establish nonspuriousness. A spurious association is one in which an association between two variables appears to be causal but can in fact be explained by some third variable. For example, we could point to the fact that older cohorts are less likely to have used marijuana. Maybe it is actually use of marijuana that leads people to be more open to legalization, not their age. This is often referred to as the third variable problem, where a seemingly true causal explanation is actually caused by a third variable not in the hypothesis. In this example, the association between age and support for legalization could be more about having tried marijuana than the age of the person.
Quantitative researchers are sensitive to the effects of potentially spurious associations. They are an important form of critique of scientific work. As a result, they will often measure these third variables in their study, so they can control for their effects. These are called control variables, and they refer to variables whose effects are controlled for mathematically in the data analysis process. Control variables can be a bit confusing, but think about it as an argument between you, the researcher, and a critic.
Researcher: “The older a person is, the less likely they are to support marijuana legalization.”
Critic: “Actually, it’s more about whether a person has used marijuana before. That is what truly determines whether someone supports marijuana legalization.”
Researcher: “Well, I measured previous marijuana use in my study and mathematically controlled for its effects in my analysis. The association between age and support for marijuana legalization is still statistically significant and is the most important association here.”
Let’s consider a few additional, real-world examples of spuriousness. Did you know, for example, that high rates of ice cream sales have been shown to cause drowning? Of course, that’s not really true, but there is a positive association between the two. In this case, the third variable that causes both high ice cream sales and increased deaths by drowning is time of year, as the summer season sees increases in both (Babbie, 2010). Here’s another good one: it is true that as the salaries of Presbyterian ministers in Massachusetts rise, so too does the price of rum in Havana, Cuba. Well, duh, you might be saying to yourself. Everyone knows how much ministers in Massachusetts love their rum, right? Not so fast. Both salaries and rum prices have increased, true, but so has the price of just about everything else (Huff & Geis, 1993).
Finally, research shows that the more firefighters present at a fire, the more damage is done at the scene. What this statement leaves out, of course, is that as the size of a fire increases so too does the amount of damage caused as does the number of firefighters called on to help (Frankfort-Nachmias & Leon-Guerrero, 2011). In each of these examples, it is the presence of a third variable that explains the apparent association between the two original variables.
In sum, the following criteria must be met for a correlation to be considered causal:
- The two variables must vary together.
- The association must be plausible.
- The cause must precede the effect in time.
- The association must be nonspurious (not due to a third variable).
Once these criteria are met, there is a nomothetic causal explanation, one that is objectively true. However, this is difficult for researchers to achieve. You will almost never hear researchers say that they have proven their hypotheses. A statement that bold implies that a association has been shown to exist with absolute certainty and that there is no chance that there are conditions under which the hypothesis would not be true. Instead, researchers tend to say that their hypotheses have been supported (or not). This more cautious way of discussing findings allows for the possibility that new evidence or new ways of examining an association will be discovered. Researchers may also discuss a null hypothesis. The null hypothesis is one that predicts no association between the variables being studied. If a researcher fails to accept the null hypothesis, she is saying that the variables in question are likely to be related to one another.
Idiographic causal explanations
If you not trying to generalize, but instead are trying to establish an idiographic causal explanation, then you are likely going to use qualitative methods, reason inductively, and engage in exploratory or descriptive research. We can understand these assumptions by walking through them, one by one.
Researchers seeking idiographic causal explanation are not trying to generalize, so they have no need to reduce phenomena to mathematics. In fact, using the language of mathematics to reduce the social world down is a bad thing, as it robs the causality of its meaning and context. Idiographic causal explanations are bound within people’s stories and interpretations. Usually, these are expressed through words. Not all qualitative studies analyze words, as some can use interpretations of visual or performance art, but the vast majority of social science studies do.
But wait, we predicted that an idiographic causal explanation would use descriptive or exploratory research. How can we build causality if we are just describing or exploring a topic? Wouldn’t we need to do explanatory research to build any kind of causal explanation? To clarify, explanatory research attempts to establish nomothetic causal explanations—an independent variable is demonstrated to cause changes a dependent variable. Exploratory and descriptive qualitative research are actually descriptions of the causal explanations established by the participants in your study. Instead of saying “x causes y,” your participants will describe their experiences with “x,” which they will tell you was caused by and influenced a variety of other factors, depending on time, environment, and subjective experience. As stated before, idiographic causal explanations are messy. The job of a social science researcher is to accurately identify patterns in what participants describe.
Let’s consider an example. What would you say if you were asked why you decided to become a social worker? If we interviewed many social workers about their decisions to become social workers, we might begin to notice patterns. We might find out that many social workers begin their careers based on a variety of factors, such as: personal experience with a disability or social injustice, positive experiences with social workers, or a desire to help others. No one factor is the “most important factor,” like with nomothetic causal explanations. Instead, a complex web of factors, contingent on context, emerge in the dataset when you interpret what people have said.
Finding patterns in data, as you’ll remember from Chapter 2, is what inductive reasoning is all about. A qualitative researcher collects data, usually words, and notices patterns. Those patterns inform the theories we use in social work. In many ways, the idiographic causal explanations created in qualitative research are like the social theories we reviewed in Chapter 2 and other theories you use in your practice and theory courses. Theories are explanations about how different concepts are associated with each other how that network of associations works in the real world. While you can think of theories like Systems Theory as Theory (with a capital “T”), inductive causality is like theory with a small “t.” It may apply only to the participants, environment, and moment in time in which the data were gathered. Nevertheless, it contributes important information to the body of knowledge on the topic studied.
Unlike nomothetic causal explanations, there are no formal criteria (e.g., covariation) for establishing causality in idiographic causal explanations. In fact, some criteria like temporality and nonspuriousness may be violated. For example, if an adolescent client says, “It’s hard for me to tell whether my depression began before my drinking, but both got worse when I was expelled from my first high school,” they are recognizing that oftentimes it’s not so simple that one thing causes another. Sometimes, there is a reciprocal association where one variable (depression) impacts another (alcohol abuse), which then feeds back into the first variable (depression) and also into other variables (school). Other criteria, such as covariation and plausibility still make sense, as the associations you highlight as part of your idiographic causal explanation should still be plausibly true and it elements should vary together.
Similarly, idiographic causal explanations differ in terms of hypotheses. If you recall from the last section, hypotheses in nomothetic causal explanations are testable predictions based on previous theory. In idiographic research, instead of predicting that “x will decrease y,” researchers will use previous literature to figure out what concepts might be important to participants and how they believe participants might respond during the study. Based on an analysis of the literature a researcher may formulate a few tentative hypotheses about what they expect to find in their qualitative study. Unlike nomothetic hypotheses, these are likely to change during the research process. As the researcher learns more from their participants, they might introduce new concepts that participants talk about. Because the participants are the experts in idiographic causal explanation, a researcher should be open to emerging topics and shift their research questions and hypotheses accordingly.
Complementary approaches to causality
Over time, as more qualitative studies are done and patterns emerge across different studies and locations, more sophisticated theories emerge that explain phenomena across multiple contexts. In this way, qualitative researchers use idiographic causal explanations for theory building or the creation of new theories based on inductive reasoning. Quantitative researchers, on the other hand, use nomothetic causal explanations for theory testing, wherein a hypothesis is created from existing theory (big T or small t) and tested mathematically (i.e., deductive reasoning). Once a theory is developed from qualitative data, a quantitative researcher can seek to test that theory. In this way, qualitatively-derived theory can inspire a hypothesis for a quantitative research project.
Two different baskets
Idiographic and nomothetic causal explanations form the “two baskets” of research design elements pictured in Figure 4.4 below. Later on, they will also determine the sampling approach, measures, and data analysis in your study.
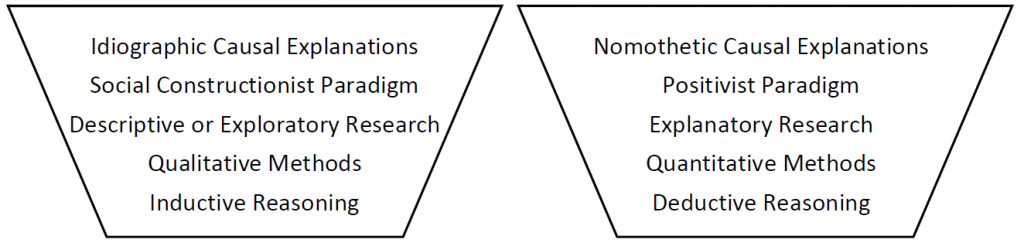
In most cases, mixing components from one basket with the other would not make sense. If you are using quantitative methods with an idiographic question, you wouldn’t get the deep understanding you need to answer an idiographic question. Knowing, for example, that someone scores 20/35 on a numerical index of depression symptoms does not tell you what depression means to that person. Similarly, qualitative methods are not often used to deductive reasoning because qualitative methods usually seek to understand a participant’s perspective, rather than test what existing theory says about a concept.
However, these are not hard-and-fast rules. There are plenty of qualitative studies that attempt to test a theory. There are fewer social constructionist studies with quantitative methods, though studies will sometimes include quantitative information about participants. Researchers in the critical paradigm can fit into either bucket, depending on their research question, as they focus on the liberation of people from oppressive internal (subjective) or external (objective) forces.
We will explore later on in this chapter how researchers can use both buckets simultaneously in mixed methods research. For now, it’s important that you understand the logic that connects the ideas in each bucket. Not only is this fundamental to how knowledge is created and tested in social work, it speaks to the very assumptions and foundations upon which all theories of the social world are built!
Key Takeaways
- Idiographic research focuses on subjectivity, context, and meaning.
- Nomothetic research focuses on objectivity, prediction, and generalizing.
- In qualitative studies, the goal is generally to understand the multitude of causes that account for the specific instance the researcher is investigating.
- In quantitative studies, the goal may be to understand the more general causes of some phenomenon rather than the idiosyncrasies of one particular instance.
- For nomothetic causal explanations, an association must be plausible and nonspurious, and the cause must precede the effect in time.
- In a nomothetic causal explanations, the independent variable causes changes in a dependent variable.
- Hypotheses are statements, drawn from theory, which describe a researcher’s expectation about an association between two or more variables.
- Qualitative research may create theories that can be tested quantitatively.
- The choice of idiographic or nomothetic causal explanation requires a consideration of methods, paradigm, and reasoning.
- Depending on whether you seek a nomothetic or idiographic causal explanation, you are likely to employ specific research design components.
Glossary
- Causality-the idea that one event, behavior, or belief will result in the occurrence of another, subsequent event, behavior, or belief
- Control variables- potential “third variables” effects are controlled for mathematically in the data analysis process to highlight the relationship between the independent and dependent variable
- Covariation- the degree to which two variables vary together
- Dependent variable- a variable that depends on changes in the independent variable
- Generalize- to make claims about a larger population based on an examination of a smaller sample
- Hypothesis- a statement describing a researcher’s expectation regarding what she anticipates finding
- Idiographic research- attempts to explain or describe your phenomenon exhaustively, based on the subjective understandings of your participants
- Independent variable- causes a change in the dependent variable
- Nomothetic research- provides a more general, sweeping explanation that is universally true for all people
- Plausibility- in order to make the claim that one event, behavior, or belief causes another, the claim has to make sense
- Spurious relationship- an association between two variables appears to be causal but can in fact be explained by some third variable
- Statistical significance- confidence researchers have in a mathematical relationship
- Temporality- whatever cause you identify must happen before the effect
- Theory building- the creation of new theories based on inductive reasoning
- Theory testing- when a hypothesis is created from existing theory and tested mathematically
4.3 Unit of analysis and unit of observation
Learning Objectives
- Define units of analysis and units of observation, and describe the two common errors people make when they confuse the two
Another point to consider when designing a research project, and which might differ slightly in qualitative and quantitative studies, has to do with units of analysis and units of observation. These two items concern what you, the researcher, actually observe in the course of your data collection and what you hope to be able to say about those observations. A unit of analysis is the entity that you wish to be able to say something about at the end of your study, probably what you’d consider to be the main focus of your study. A unit of observation is the item (or items) that you actually observe, measure, or collect in the course of trying to learn something about your unit of analysis.
In a given study, the unit of observation might be the same as the unit of analysis, but that is not always the case. For example, a study on electronic gadget addiction may interview undergraduate students (our unit of observation) for the purpose of saying something about undergraduate students (our unit of analysis) and their gadget addiction. Perhaps, if we were investigating gadget addiction in elementary school children (our unit of analysis), we might collect observations from teachers and parents (our units of observation) because younger children may not report their behavior accurately. In this case and many others, units of analysis are not the same as units of observation. What is required, however, is for researchers to be clear about how they define their units of analysis and observation, both to themselves and to their audiences.
More specifically, your unit of analysis will be determined by your research question. Your unit of observation, on the other hand, is determined largely by the method of data collection that you use to answer that research question. We’ll take a closer look at methods of data collection later on in the textbook. For now, let’s consider again a study addressing students’ addictions to electronic gadgets. We’ll consider first how different kinds of research questions about this topic will yield different units of analysis. Then, we’ll think about how those questions might be answered and with what kinds of data. This leads us to a variety of units of observation.
If we were to explore which students are most likely to be addicted to their electronic gadgets, our unit of analysis would be individual students. We might mail a survey to students on campus, and our aim would be to classify individuals according to their membership in certain social groups in order to see how membership in those classes correlated with gadget addiction. For example, we might find that majors in new media, men, and students with high socioeconomic status are all more likely than other students to become addicted to their electronic gadgets. Another possibility would be to explore how students’ gadget addictions differ and how are they similar. In this case, we could conduct observations of addicted students and record when, where, why, and how they use their gadgets. In both cases, one using a survey and the other using observations, data are collected from individual students. Thus, the unit of observation in both examples is the individual.
Another common unit of analysis in social science inquiry is groups. Groups of course vary in size, and almost no group is too small or too large to be of interest to social scientists. Families, friendship groups, and group therapy participants are some common examples of micro-level groups examined by social scientists. Employees in an organization, professionals in a particular domain (e.g., chefs, lawyers, social workers), and members of clubs (e.g., Girl Scouts, Rotary, Red Hat Society) are all meso-level groups that social scientists might study. Finally, at the macro-level, social scientists sometimes examine policies, citizens of entire nations, or residents of different continents or other regions.
A study of student addictions to their electronic gadgets at the group level might consider whether certain types of social clubs have more or fewer gadget-addicted members than other sorts of clubs. Perhaps we would find that clubs that emphasize physical fitness, such as the rugby club and the scuba club, have fewer gadget-addicted members than clubs that emphasize cerebral activity, such as the chess club and the women’s studies club. Our unit of analysis in this example is groups because groups are what we hope to say something about. If we had instead asked whether individuals who join cerebral clubs are more likely to be gadget-addicted than those who join social clubs, then our unit of analysis would have been individuals. In either case, however, our unit of observation would be individuals.
Organizations are yet another potential unit of analysis that social scientists might wish to say something about. Organizations include entities like corporations, colleges and universities, and even nightclubs. At the organization level, a study of students’ electronic gadget addictions might explore how different colleges address the problem of electronic gadget addiction. In this case, our interest lies not in the experience of individual students but instead in the campus-to-campus differences in confronting gadget addictions. A researcher conducting a study of this type might examine schools’ written policies and procedures, so her unit of observation would be documents. However, because she ultimately wishes to describe differences across campuses, the college would be her unit of analysis.
In sum, there are many potential units of analysis that a social worker might examine, but some of the most common units include the following: individuals, groups, and organizations.
Research question | Unit of analysis | Data collection | Unit of observation | Statement of findings |
Which students are most likely to be addicted to their electronic gadgets? | Individuals | Survey of students on campus | Individuals | New Media majors, men, and students with high socioeconomic status are all more likely than other students to become addicted to their electronic gadgets. |
Do certain types of social clubs have more gadget-addicted members than other sorts of clubs? | Groups | Survey of students on campus | Individuals | Clubs with a scholarly focus, such as social work club and the math club, have more gadget-addicted members than clubs with a social focus, such as the 100-bottles-of- beer-on-the-wall club and the knitting club. |
How do different colleges address the problem of electronic gadget addiction? | Organizations | Content analysis of policies | Documents | Campuses without strong computer science programs are more likely than those with such programs to expel students who have been found to have addictions to their electronic gadgets. |
Note: Please remember that the findings described here are hypothetical. There is no reason to think that any of the hypothetical findings described here would actually bear out if tested with empirical research. |
One common error people make when it comes to both causality and units of analysis is something called the ecological fallacy. This occurs when claims about one lower-level unit of analysis are made based on data from some higher-level unit of analysis. In many cases, this occurs when claims are made about individuals, but only group-level data have been gathered. For example, we might want to understand whether electronic gadget addictions are more common on certain campuses than on others. Perhaps different campuses around the country have provided us with their campus percentage of gadget-addicted students, and we learn from these data that electronic gadget addictions are more common on campuses that have business programs than on campuses without them. We then conclude that business students are more likely than non-business students to become addicted to their electronic gadgets. However, this would be an inappropriate conclusion to draw. Because we only have addiction rates by campus, we can only draw conclusions about campuses, not about the individual students on those campuses. Perhaps the social work majors on the business campuses are the ones that caused the addiction rates on those campuses to be so high. The point is we simply don’t know because we only have campus-level data. By drawing conclusions about students when our data are about campuses, we run the risk of committing the ecological fallacy.
On the other hand, another mistake to be aware of is reductionism. Reductionism occurs when claims about some higher-level unit of analysis are made based on data from some lower-level unit of analysis. In this case, claims about groups or macro-level phenomena are made based on individual-level data. An example of reductionism can be seen in some descriptions of the civil rights movement. On occasion, people have proclaimed that Rosa Parks started the civil rights movement in the United States by refusing to give up her seat to a white person while on a city bus in Montgomery, Alabama, in December 1955. Although it is true that Parks played an invaluable role in the movement, and that her act of civil disobedience gave others courage to stand up against racist policies, beliefs, and actions, to credit Parks with starting the movement is reductionist. Surely the confluence of many factors, from fights over legalized racial segregation to the Supreme Court’s historic decision to desegregate schools in 1954 to the creation of groups such as the Student Nonviolent Coordinating Committee (to name just a few), contributed to the rise and success of the American civil rights movement. In other words, the movement is attributable to many factors—some social, others political and others economic. Did Parks play a role? Of course she did—and a very important one at that. But did she cause the movement? To say yes would be reductionist.
It would be a mistake to conclude from the preceding discussion that researchers should avoid making any claims whatsoever about data or about relationships between levels of analysis. While it is important to be attentive to the possibility for error in causal reasoning about different levels of analysis, this warning should not prevent you from drawing well-reasoned analytic conclusions from your data. The point is to be cautious and conscientious in making conclusions between levels of analysis. Errors in analysis come from a lack of rigor and deviating from the scientific method.
Key Takeaways
- A unit of analysis is the item you wish to be able to say something about at the end of your study while a unit of observation is the item that you actually observe.
- When researchers confuse their units of analysis and observation, they may be prone to committing either the ecological fallacy or reductionism.
Glossary
- Ecological fallacy- claims about one lower-level unit of analysis are made based on data from some higher-level unit of analysis
- Reductionism- when claims about some higher-level unit of analysis are made based on data at some lower-level unit of analysis
- Unit of analysis- entity that a researcher wants to say something about at the end of her study
- Unit of observation- the item that a researcher actually observes, measures, or collects in the course of trying to learn something about her unit of analysis
4.4 Mixed methods
Learning Objectives
- Define sequence and emphasis and describe how they work in qualitative research
- List the five reasons why researchers use mixed methods
So far in this textbook, we have talked about quantitative and qualitative methods as an either/or choice—you can choose quantitative methods or qualitative methods. However, researchers often use both methods inside of their research projects. Take for example a recent study of the possibility of having optometrists refer their older patients to group exercise programs (Miyawaki, Mauldin, & Carman, 2019). In this study, a short, written survey was distributed to optometrists across Texas. The survey asked closed-ended questions about their practice, knowledge about fall prevention, and attitudes about prescribing group exercise programs to their patients. While the study could have just surveyed optometrists for a descriptive quantitative analysis, it was designed to capture more rich details about the perspectives of older optometry patients through conducting focus groups with them. In the focus groups, the older adults were asked about their perceptions of being prescribed group exercise classes by their optometrist. The study used both qualitative and quantitative methods, or a mixed methods design.
Sequence and emphasis
There are many different mixed methods designs, each with their own strengths. However, a more simplified synthesis of mixed methods approaches is provided by Engel and Schutt (2016) using two key terms. Sequence refers to the order that each method is used. Researchers can use both methods at the same time or concurrently. Or, they can use one and then the other, or sequentially. The optometry study used a concurrent design in which data were collected and analyzed concurrently. The researchers could have used a sequential design in which one part of the study was conducted first, data analyzed, and then used to inform the researchers about how to conduct the second part of the study.
The other key term in mixed methods research is emphasis. In some studies, the qualitative data may be the most important, with the quantitative data providing secondary or background information. In this case qualitative methods are prioritized. Other times, however, quantitative methods are emphasized. In these studies, qualitative data are used mainly to provide context for the quantitative findings. For example, demonstrating quantitatively that a particular therapy works is important. By adding a qualitative component, researchers could find out how the participants experienced the intervention, how they understood its effects, and the meaning it had on their lives. These data would add depth and context to the findings of the study and allow researchers to improve the therapeutic technique in the future.
A similar practice is when researchers use qualitative methods to solicit feedback on a quantitative scale or measure. The experiences of individuals allow researchers to refine the measure before they do the quantitative component of their study. Finally, it is possible that researchers are equally interested in qualitative and quantitative information. In studies of equal emphasis, researchers consider both methods as the focus of the research project.
Why researchers use mixed methods
Mixed methods research is more than just sticking an open-ended question at the end of a quantitative survey. Mixed methods researchers use mixed methods for both pragmatic and synergistic reasons. That is, they use both methods because it makes sense with their research questions and because they will get the answers they want by combining the two approaches.
Mixed methods also allows you to use both inductive and deductive reasoning. As we’ve discussed, qualitative research follows inductive logic, moving from data to empirical generalizations or theory. In a mixed methods study, a researcher could use the results from a qualitative component to inform a subsequent quantitative component. The quantitative component would use deductive logic, using the theory derived from qualitative data to create and test a hypothesis. In this way, mixed methods use the strengths of both research methods, using each method to understand different parts of the same phenomenon. Quantitative allows the researcher to test new ideas. Qualitative allows the researcher to create new ideas.
With these two concepts in mind, we can start to see why researchers use mixed methods in the real world. Mixed methods are often to initiate ideas with one method to study with another. For example, researchers could begin a mixed methods project by using qualitative methods to interview or conduct a focus group with participants. Based on their responses, the researchers could then formulate a quantitative project to follow up on the results.
In addition to providing information for subsequent investigation, using both quantitative and qualitative information provides additional context for the data. For example, in the optometry/group exercise study, most optometrists expressed that they would be willing to prescribe exercise classes to their patients. In the focus groups, the patients were able to describe how they would respond to receiving a prescription from their optometrist and the barriers they faced to going to exercise classes. The context provided by the qualitative focus group data provides important context for practitioners building clinical-community partnerships to help prevent falls among older adults.
Finally, another purpose of mixed methods research is corroborating data from both quantitative and qualitative sources. Ideally, your qualitative and quantitative results should support each other. For example, if interviews with participants showed a relationship between two concepts, that relationship should also be present in the qualitative data you collected. Differences between quantitative and qualitative data require an explanation. Perhaps there are outliers or extreme cases that pushed your data in one direction or another, for example.
In summary, these are a few of the many reasons researchers use mixed methods. They are summarized below:
- Triangulation or convergence on the same phenomenon to improve validity
- Complementarity, which aims to get at related but different facets of a phenomenon
- Development or the use of results from one phase or a study to develop another phase
- Initiation or the intentional analysis of inconsistent qualitative and quantitative findings to derive new insights
- Expansion or using multiple components to extend the scope of a study (Burnett, 2012, p. 77).
A word of caution
The use of mixed methods has many advantages. However, researchers should approach mixed methods with caution. Conducting a mixed methods study may mean doubling or even tripling your work. You must conceptualize how to use one method, another method, and how they fit together. This may mean operationalizing and creating a questionnaire, then writing an interview guide, and thinking through how the data on each measure relate to one another—more work than using one quantitative or qualitative method alone. Similarly, in sequential studies, the researcher must collect and analyze data from one component and then conceptualize and conduct the second component. This may also impact how long a project may take. Before beginning a mixed methods project, you should have a clear vision for what the project will entail and how each methodology will contribute to that vision.
Key Takeaways
- Mixed methods studies vary in sequence and emphasis.
- Mixed methods allow the research to corroborate findings, provide context, follow up on ideas, and use the strengths of each method.
Glossary
- Emphasis- in a mixed methods study, refers to the priority that each method is given
- Sequence- in a mixed methods study, refers to the order that each method is used, either concurrently or sequentially